Abstract
Purpose
This research aims to understand the dynamics that drive consumer engagement of multinational brands' social media posts on platform X, formerly known as Twitter. Taking the emotional tone of posts into account, the effect of vivid, interactive, informative, entertaining and practical features of posts on consumer interactions are evaluated across English- and Turkish-speaking markets.
Methodology
Inspired by the conceptual framework proposed in previous literature, features were extracted computationally using natural language processing from platform X posts of 33 Fortune 500 brands from various industries from June 2016 to June 2021. Following evaluation of regression models on alternative distributions of the dependent variable, which is total number of likes, shares and comments, random subspace regression using bootstrap resampling was applied to calculate an importance score and evaluate the effect of features.
Findings
Consumers in English- and Turkish-speaking markets perceive and engage with content differently. While informative and entertaining posts resonate more with English speakers, emotions play a broader role for Turkish speakers. English-speaking audience prefers happy and vivid daytime messages with questions, while Turkish-speaking audience is drawn to angry messages, lean toward nighttime posts.
Originality
This research is a pioneer to evaluate the factors that influence brands' platform X post engagements across markets of different cultural orientation. Beyond assessing the distinctions in brand post elements, the role of emotional content in brand messages were also analyzed across English- and Turkish-speaking markets.
Keywords
Citation
Tanaltay, A., Ozturkcan, S. and Kasap, N. (2024), "Decoding digital engagement: a comparative analysis of English and Turkish brand post popularity dynamics on platform X", Journal of Research in Interactive Marketing, Vol. ahead-of-print No. ahead-of-print. https://doi.org/10.1108/JRIM-10-2023-0368
Publisher
:Emerald Publishing Limited
Copyright © 2024, Tanaltay Altug, Ozturkcan Selcen and Kasap Nihat
License
Licensed re-use rights only
1. Introduction
In today's digitally interconnected world, brands are in a constant quest for attention and the establishment of meaningful connections with their target audience to promote their products and services (Lee and Kahle, 2016). Social media platforms have risen as influential tools for online brand communication, offering a unique space where consumers can engage, express their opinions, create communities and share information (Delia and Armstrong, 2015). Through interactions on social media, consumers shape their perceptions of a brand and build relationships (Hudson et al., 2015). Due to an established strong relationship in online media, a brand may eventually become irreplaceable to consumers (Ekinci et al., 2004). Successful online marketing efforts are known to influence consumer engagement, encompassing the spread of positive word-of-mouth and endorsements for the brand (Edelman, 2010). With the unprecedented growth in user-generated content and feedback, examining the factors contributing to increased consumer engagement with a brand's social media posts can uncover valuable lessons for business.
Numerous studies have been undertaken on evaluating the effects of factors connected to a brand's social media post's vivid, practical, interactive, informative and entertaining features, using likes, comments and shares as measures of brand post popularity and consumer engagement (Schultz, 2017) The content factors, including emotional sentiment, format and interestingness of brand messages, are anticipated to influence consumers' reactions, comments and shares. These measures are considered relevant indicators of consumer engagement, which results in brand loyalty and purchase intentions (Barger et al., 2016). However, despite the growing research on social media posts' global attractiveness to consumers, three issues need to be investigated further:
First, one issue that hasn't received sufficient examination among brand post features is the influence of emotional appeal, which pertains to the emotions a social media post aims to evoke. In many instances, this has been oversimplified to merely evaluating the effect of positive or negative emotions. Emotions have a significant impact on the overall consumer experience (Laverie et al., 1993) and can significantly affect word-of-mouth communication and brand loyalty (Crosby and Johnson, 2007). Hence, there is a need to expand the spectrum of emotions and comprehend the effects of more specific appeals, such as happiness, sadness, anger or fear. This expanded understanding is crucial for effectively managing the emotional tone in social media messages.
The second point to consider is that research into the global appeal of social media posts to consumers and the influence of cultural variations on consumer responses to branding communication remains limited. Cultural aspects related to individualism/collectivism, uncertainty avoidance, power distance and long-term orientation (Hofstede, 2011) have been demonstrated to be valuable in understanding the impact of social media campaigns in diverse cultural contexts (Lin et al., 2017). Depending on one's cultural perspective, consumers may interpret brand messages differently. Therefore, culture emerges as a significant factor that managers should consider when customizing their social media campaigns for consumers from distinct cultural backgrounds.
Third, when it comes to the impacts of various factors, there have been conflicting findings. Previous research on brand post popularity has predominantly used ordinary least squares (OLS) regression to analyze post interactions and draw conclusions from confidence intervals and p-values of independent variables under concern. However, the validity of inferences made using OLS regression parameter estimates relies on certain assumptions. These assumptions include assuming a linear relationship between the dependent and independent variables, no correlation among the independent variables and normally distributed error terms with consistent variance (Berry, 1993). Though challenging to verify, even slight violations of these assumptions can result in misleading estimates and statistics. Additionally, the models proposed may not exhibit the same level of explanatory power when applied to data beyond the specific sample under examination. Consequently, a more adaptable approach is necessary to explore the influential features and their effects for improved generalizability.
Taking these issues into account, this research delves into the dynamics of brand post popularity. It explores the various components that propel consumer engagement and, in the end, influence the effectiveness of brand communication efforts in social media platform X (formerly known as Twitter), seeking answer to the following Research Question (RQ):
RQ1. What are the most influential factors explaining brand post popularity across English- and Turkish-speaking markets on platform X?
To address the first issue, this study seeks to delve into the underlying mechanisms and analyze the impacts of various factors, including vividness, practicality, interactivity, information and entertainment features, as well as distinct emotions such as happiness, surprise, anger, fear, disgust and sadness. For the second issue, which pertains to the cultural perspective, the research examines platform X posts from multinational brands. Posts in English represent markets characterized by more individualistic cultures, while posts in Turkish represent markets with a more collectivist orientation. Finally, to tackle the third issue, the study evaluates multiple regression models under various target variable distributions. Following the selection of the most suitable model, Monte Carlo simulations are employed on random subsets of features and data to mitigate the impact of any violations of regression assumptions for inference.
This research attempts to shed light on the complex link that exists between brands, consumers and the social media domain, giving significant information for marketers and scholars alike, making several significant theoretical and managerial contributions:
Introducing a novel bootstrap approach for assessing and scoring the impacts of factors associated with brand post popularity.
Gaining insights into the influence of culture on these factors through the development and comparison of statistical models constructed separately for English and Turkish datasets. This study is pioneering in its focus on the cultural aspect of brand post popularity.
Not only evaluating cross-cultural distinctions in terms of the vividness, practicality, interactivity, information and entertainment aspects of brand platform X posts but also incorporating the emotional content of messages as an additional factor influencing brand post popularity. This represents the first study to analyze how emotions conveyed by brands in their social media posts affect the success of brand messages comparatively across different cultures.
The rest of this paper is organized as follows: It begins with a review of the theoretical background concerning brand and consumer engagement on social media and discusses the conceptual framework that inspired this study. This is followed by a detailed description of the research methodology and the presentation of the results. The final section addresses the theoretical and managerial implications, outlines the limitations of the study and suggests directions for future research.
2. Theoretical background and conceptualization
2.1 Social media and consumer engagement
Scholars and business professionals alike are keen to understand the ways in which social media can positively or negatively affect the relationship between consumers and brands. However, according to Schultz and Peltier (2013), the majority of social media marketing efforts consist of sharing sales promotions with consumers who are already engaged with the brand. The authors suggest that marketers should explore strategies to leverage social media for building enduring brand relationships instead of simply using these platforms to boost short-term sales.
Taking up with the future research directions of Schultz and Peltier (2013), Barger et al. (2016) proposed a framework to define, conceptualize and categorize the antecedents and consequences concerning social media activities. Accordingly, emotional sentiments, vividness, entertainment and informativeness are considered important factors in branded content that contribute to consumer engagement. Due to the interactivity and collaborativeness of social media, factors leading to and resulting from brand engagement could be analyzed through essential social media metrics like likes, ratings, comments and shares (Barger and Labrecque, 2013). Engaged use of social media may lead to several beneficial consequences for brands, including enhanced customer satisfaction, loyalty, retention, lifetime value, wallet share and profitability (Cummins et al., 2014).
Researching the content factors that impact social media interaction is crucial due to the significant increase in social media messaging, both locally and globally. Emerging social media platforms have presented abundant research prospects, enabling the examination of how diverse messaging strategies impact customer engagement across various social media platforms (Barger et al., 2016). This study focuses on platform X and aims to address how content factors effect consumer engagement, which is in the context of social media brand messages conceptualized as “Brand Post Popularity” (De Vries et al., 2012). Prior studies demonstrated that external factors as the cultural background of the consumers, beyond the content features, have the ability to influence the level of consumer engagement with brands on social media (Lin et al., 2017). In societies characterized by high individualism, people prioritize their personal goals over those of their groups and rely on their personal attitudes rather than group norms to guide their behavior. On the other hand, collectivist societies consist of individuals who are deeply interconnected within their groups, prioritize the goals and norms of these groups and act in a way that benefits the community. Exploring how these cultural dimensions influence online consumer engagement is crucial for enabling brands to more effectively navigate the complexities of global digital marketing strategies. Currently, there exists a gap of comprehensive research that integrate the cultural dimensions to understand their combined impact on consumer engagement (Vander Schee et al., 2020). This study explicitly examines the impact of culture on brand post engagements by comparing the attitudes in English- and Turkish-speaking markets.
2.2 Brand post popularity
The rapid growth of brands' social media advertising efforts has garnered significant attention within the research community, leading to numerous studies in this domain. The first comprehensive study was conducted by De Vries et al. (2012), drawing inspiration from prior research on effectiveness of banner advertising. Brand post popularity is generally referred to the number of consumer engagements of a brand's social media messages and has typically been operationalized by quantifying likes, comments and shares. While some prior studies have employed distinct models to explain likes, comments and shares individually (Antoniadis et al., 2019; Banerjee and Chua, 2019; Schultz, 2017), others favored a weighted combination of number of likes, shares and comments as the dependent variable (Karpinska-Krakowiak and Modlinski, 2020). Facebook has been the most intensively studied platform, followed by Instagram (Geurin and Burch, 2017; Mazloom et al., 2016; Yu and Sun, 2019), with X (Twitter) receiving less attention (Zadeh and Sharda, 2014).
Common factors of brand post popularity analyzed in previous literature and this study include vividness, interactivity, informativeness, entertaining content and emotional appeal in social media posts:
Vividness pertains to the extent to which a post stimulates users' visual senses. Posts with images or videos are considered more vivid, and previous studies, such as De Vries et al. (2012) and Banerjee and Chua (2019), have found that vivid posts attract more user interactions.
Interactivity measures the degree to which a post encourages user action or allows them to influence communication. Features like links, hashtags, mentions, questions or polls define the interactivity of a post. (De Vries et al., 2012) and others have observed that higher interactivity leads to more interactions, although the relationship may vary in different contexts.
Practicality assesses the accessibility and efficiency of delivering the brand's message. Factors like post length, timing and post duration impact a post's practicality. Research findings differ concerning the effects of these practicality, while some suggests that posts at unusual hours can boost engagement (Sabate et al., 2014).
Information relates to the extent to which a post provides detailed information about various topics. While some studies as Mazloom et al., 2016's have found a positive effect of informative content, while others such as De Vries et al. (2012) and Antoniadis et al. (2019) have reported no significant relationship.
Entertaining posts are those that reflect fun, humor or excitement. De Vries et al. (2012) suggested that entertaining content might not be directly related to a brand's focus, potentially attracting less attention from consumers. However, recent studies, including Karpinska-Krakowiak and Modlinski (2020), have found that humor can increase post interactions.
Emotional appeal involves targeting specific emotions through a post. Swani et al. (2013) and Pinto and Yagnik (2017) showed that including emotional sentiments in brand posts increased user attention and interactions. This study takes a different approach, representing message appeal using seven categories derived from the textual content of posts, including basic emotions like happiness, surprise, anger, sadness, disgust, fear and neutrality.
Although not empirically tested, Lin et al. (2017) argued that social media practices may not be equally effective in various regional markets. They suggested adapting social media practices based on Hofstede's cultural dimensions theory, encompassing individualism/collectivism, uncertainty avoidance, power distance and long-term orientation (Hofstede et al., 2010). Such cultural dimensions could be valuable for customizing social media practices for different cultural audiences, as consumers from diverse societies may exhibit distinct attitudes and behaviors. As shown by Izogo and Mpinganjira (2020), inspirational content, whether utilitarian or hedonic, has a variably positive impact on customer inspiration, with hedonic content having a stronger influence than utilitarian content. Consumers with a collectivist orientation are more easily inspired than ones from individualistic cultures. Consequently, consumers' cultural background not only stimulates customer engagement behaviors and the intent to purchase but also acts as a mediator, channeling the inspiration drawn from content to influence consumer engagement behavior and purchase decisions. The majority of previous research predominantly focused on English-language datasets, resulting in contradictory findings regarding the impact of specific variables and highlighting the necessity for validation via diverse approaches across varying contexts. The current study seeks to address the empirical gap in earlier research by investigating how common antecedents and features impact consumer interactions differently across Turkish-speaking (collectivist) and English-speaking (individualistic) consumers.
2.3 Conceptual framework
This study aims to investigate the key factors influencing the popularity of brand posts on platform X from a cross-cultural perspective. The popularity of social media posts by brands is examined through a conceptual framework (Figure 1) that is based on prior model proposed by De Vries et al. (2012) and modified by Schultz (2017) and Antoniadis et al. (2019).
The impact of these factors is assessed not only by their effect sizes and statistical significance but also by how they vary across English-speaking (individualistic) and Turkish-speaking (collectivist) markets. The popularity of brand posts, the dependent variable, is measured by the total number of likes, shares and comments, across six categories of factors: vividness, interactivity, practicality, informative content, entertaining content and emotional appeal. Controlling for the number of followers and the industry of the brand posts is essential, as these factors directly affect post interactions. To evaluate the differences in consumer interactions between English and Turkish markets, two sets of parameter coefficient estimates are computed for each of the two markets, based on the factors within the conceptual framework.
In this research, the primary dependent variable is the Total Number of Interactions, which is the sum of likes, shares and comments. Most previous studies have used OLS regression to analyze the conceptual model, but this approach requires certain assumptions to be met, such as linear relationships among variables, no correlation among independent variables and normally distributed error terms with constant variance. Multicollinearity and omitted variables can complicate coefficient interpretation and model stability. Additionally, heteroscedasticity may occur when these assumptions are violated. In order to address these challenges, bootstrap Monte Carlo simulations were employed to build multiple models on resampled data, providing more reliable estimates of regression coefficients, confidence intervals and p-values for statistical inference.
3. Methodology
The study's research design is illustrated in Figure 2. Following data collection, variables were operationalized based on the reliability criteria outlined in previous brand post popularity research. Structural features of post content were extracted computationally, and emotions were extracted using natural language processing (NLP). Subsequently, the goodness-of-fit of OLS, Poisson and negative binomial (NB) regression models were assessed the relation of these features to the total number of post interactions. Additionally, random subspace regression with bootstrap resampling was employed on the most appropriate regression model to determine importance scores, coefficient estimates, confidence intervals and p-values for the variables within the regression model.
3.1 Data
This study focuses on platform X posts by brands and the consumer interactions they generate. Moreover, to bridge the cultural gap in earlier studies English language was chosen to represent markets characterized by more individualistic cultures, while the Turkish language was chosen to represent markets characterized by less individualism and more collectivism (Hofstede et al., 2010).
The study's sample consists of platform X posts from 33 Fortune 500 brands from various industries, including FMCG, Fast Food, Technology, Automotive, Apparel, Retail, Finance and Logistics. These brands actively maintain X accounts in both Turkish and English languages (for list of brands in sample, please see Appendix 1). Data collection was facilitated using X's former Academic API and Java programming language. All posts made by these brands from June 2016 to June 2021 were collected and subjected to analysis. Consequently, a total of 37,825 Turkish brand posts and 571,561 English brand posts were collected (for the number of posts, followers and total interactions by industry, please see Appendix 2).
As also noted by previous research, a fair amount of positive correlation existed among followers and interactions. Moreover, brand posts and interactions varied significantly by industry. As a result of their apparent effects, the number of followers and industry were required to be controlled in the statistical models. The total number of interactions, along with the numeric independent variables, exhibited a highly skewed and asymmetric distribution in both the Turkish and English datasets. Furthermore, there was a noticeable degree of multicollinearity among the independent variables in both language datasets. Therefore, in devising statistical models for brand post popularity, we considered relevant approaches to address distribution skewness, account for nonlinear relationships and mitigate the confounding effects of independent variables.
3.2 Operationalization of variables
While the modeling approach shared similarities with prior research, there was a difference in the operationalization of “vividness” and “interactivity” compared to previous studies where these factors were categorized as low, medium or high (De Vries et al., 2012). In this study, “vividness” category was assessed by counting the quantity of emojis, images and videos within a message, and “interactivity” of posts was evaluated by counting the number of hashtags, links, mentions and the presence of questions or polls in their content (for the quantitative representation of features by feature categories, please see Appendix 3).
All variables were extracted computationally from the payload of messages provided by platform X API, except number of emojis and the emotional content which were further extracted by NLP. “Emotional appeal” was represented through six binary variables, each corresponding to different emotions: “happy,” “surprise,” “sad,” “anger,” “disgust” and “fear.” “Neutrality” serves as the base variable for emotional appeal.
In terms of control variables, the “industry” category was represented by seven binary variables, including “Logistics,” “Food Service,” “Personal Care,” “Retail,” “Financial Services,” “FMCG” and “Automotive,” with “Technology” as the base category. Additionally, “Followers Count” served as the second control variable, which was a discrete numerical variable indicating the number of followers that the brand(s) had at the time the post was downloaded.
3.3 Method
Schultz (2017), Antoniadis et al. (2019) and Banerjee and Chua (2019) employed OLS regression to model natural log-transformed likes, shares and comments. As the target variables are discrete and not distributed normally, in Swani et al., (2013), the relationship was explained with Poisson regression (PR) and more recently in Swani and Milne (2017), by NB regression models.
In order to identify the most influential features and their respective impacts, in alignment with prior research, we utilized the linear relationship depicted in Equation 1 to model brand post popularity for both English and Turkish languages:
i denotes language, i ∈{English, Turkish} and yi = sharesi + likesi + commentsi;
βi represents the vector of regression coefficients for language i;
Xi is the matrix of values of independent variables in data;
∈i is the vector of error terms for language i.
Subsequently, the linear relationship outlined in Equation 1 is employed to evaluate goodness-of-fit of OLS, PR and NB regression models. Regarding the OLS regression model, dependent variable was natural log transformed. As OLS model demonstrated a satisfactory ability to account for the skewness of distributions for both languages, it was chosen for further examination of the impact of variables on consumer interactions (please see Appendix 4 for mathematical descriptions and evaluation of models).
Concerning the dataset of brands, the interactions exhibited a skewed distribution, and the relationship between independent and dependent variables were nonlinear. Moreover, fair amount of multicollinearity existed among independent variables. Thus, inspired by Wichitaksorn et al. (2023a), random subspace regression using the bootstrap method was employed to attain reliable estimates of coefficients, their associated confidence intervals, p-values and an importance score for each coefficient concerning the given features and conceptual model (please see Appendix 5 for the detailed description of the procedure). The lowest root mean square error (RMSE) was achieved when the bootstrap cycle was run for 1,000 iterations, a marked improvement over feature subset selection via exhaustive search, which would require exploring 229 alternative combinations, considering that 29 features were under consideration for the linear model.
4. Results
The average importance scores resulting from 1,000 random subspace bootstrap iterations on Turkish and English datasets are shown in Figure 3 (for estimates of importance scores, please see Appendix 6). Notably, control variables, including the number of followers and the industry of each brand account, exhibited the highest average importance for Turkish data, registering at 0.159. In contrast, for English data, these control variables constituted the second most influential group with an average importance score of 0.197, following the information variable. The average scores suggested that both industry and the number of followers significantly contributed to explaining engagement and interactions on X in both languages. Concerning Turkish post engagements, all categories, except control variables, hovered around an average importance score of 0.12, indicating a similar level of significance. Conversely, concerning English data, the information and entertainment categories scored notably higher, with scores of 0.243 and 0.184, respectively. This implies that within the information and entertainment categories, the variables held relatively more importance in driving interactions on platform X, particularly in the English context. Vividness, emotional appeal, interactivity and practicality features exhibited similar rankings in terms of their average importance scores for both languages, fluctuating around 0.12 for Turkish data and 0.16 for English data. However, vividness demonstrated relatively greater importance for English interactions. Finally, regarding the Emotion category, importance scores of 0.120 and 0.160 were observed for Turkish and English data, respectively. This indicated that within the emotion group, this group held relatively more importance in driving X post interactions, particularly in the English-speaking context. It suggested that evoking emotional responses through content may exert a notable influence on driving engagement and interactions on platform X among English-speaking consumers.
The confidence interval estimations for the variables following the comprehensive bootstrap procedure are presented in Figure 4 (for point estimates, please see Appendix 3). Concerning the “Industry” variables, in the English dataset, both the Logistics and Food Service industries had a significant impact, whereas in the Turkish dataset, all control variables except Financial Services were statistically significant. Naturally, an increase in the number of followers was associated with higher mean post interactions for both languages. Interestingly, the Food Service industry had divergent effects in Turkish and English data, displaying a negative effect in the Turkish dataset (mean = −1.211) and a positive effect in the English dataset (mean = 2.035).
Regarding the Practicality of X brand posts, messages posted during the daytime tended to reduce mean post interactions in Turkish, where this effect was positive in the English data. For both languages, messages posted during weekdays did not have a significant impact on post interactions. Text length exhibited different patterns in Turkish and English data. In the Turkish dataset, it had a negative effect with a mean of −0.916, while in the English dataset the effect was not significant. “Holiday” showed a positive coefficient for English and negative for Turkish, indicating that tweets posted during holidays received higher interactions for English data. Lastly, the variable “Position” had a negligible effect in the Turkish data but a positive effect in the English data, indicating that messages staying at the top position were more likely to receive likes, shares or comments in English.
Among the variables in the Interactivity category, both the number of links in Turkish and Questions in English had a significantly negative effect on mean post interactions. However, all other variables within this category exhibited negligible effects in both the Turkish and English datasets. Regarding the “Question” variable in English, the results were consistent with previous research, estimating a negative effect of questions on post interactions.
Concerning Vividness features, they did not significantly impact mean interactions in Turkish data. However, in the English data, positive coefficients and p-values for images and emojis suggested that incorporating vivid or lively elements in the content may enhance engagement and interactions on platform X, particularly among English-speaking consumers.
Within the Emotion category, both happy and sad messages significantly increased interactions, while the effect was negative for posts with an angry emotional appeal in English messages. In the Turkish data, a similar effect was observed for sad emotional appeal; however, happy posts did not have a significant effect on mean interactions. In contrast to English posts, messages containing an angry tone were more likely to increase mean interactions in Turkish data. Disgust, fear and surprise emotions in brand posts for both languages had wide confidence intervals intersecting zero, indicating that we don't have enough evidence in data to show any relationship to mean interactions.
Messages mentioning Information about their products and services had a positive effect on post interactions for both languages. However, the variable held relatively more importance in the English context, suggesting that focusing on informative content may lead to higher engagement and interactions on X. Entertainment on the other hand exhibited a positive effect in both the Turkish and English data. However, the effect was not significant in Turkish data. This suggested that focusing on entertaining content may be influential in capturing attention and generating interactions on X, particularly among English-speaking consumers.
5. Discussion and implications
5.1 Discussion
The objective of this research was to comprehend the factors that influence the level of customer engagement with social media posts of multinational brands on platform X, previously referred to as Twitter. It specifically examined the structural and textual characteristics of select brands' posts, which were categorized into vividness, practicality, interactivity, information, entertainment and emotional appeal (including happiness, anger, sadness, surprise, fear and disgust). An enhanced conceptual model was created based on previous research to quantify the popularity of brand posts using metrics such as total likes, shares and comments. As a frontier in cross-cultural research, the study focuses on comparing the posts of multinational brands on platform X in both English and Turkish. To assess and determine their significance, a bootstrap resampling method utilizing random subspace features was introduced. These results offer valuable insights not only into the varying significance of factors influencing X's post interactions in different language contexts but also into the distinctions and commonalities in how these factors impact outcomes in the Turkish and English datasets. The differences in importance scores, the directions of their effects and the significance values underscore potential disparities in consumer preferences and engagement drivers across different markets. This study presents a novel scoring method for factors that may be associated with the popularity of brand messages.
Regarding the control variables, the pronounced difference in the importance of followers count between Turkish- (0.3648) and English-speaking (0.2861) markets underscored the heightened value placed on community and social networks in Turkish-speaking audiences. This aligned with the collectivist cultural orientation, which emphasizes group relationships and loyalty. In contrast, the English-speaking market, which often reflects more individualistic cultures, showed a diversified engagement pattern where content's informational value (0.2439 in English vs 0.1217 in Turkish) played a more significant role, suggesting a preference for content that enhances individual knowledge and personal benefit.
The variability in emotional content's impact, with emotions like anger and disgust having higher importance scores in the English context, points to cultural differences in emotional expressiveness and its acceptance on social media. The positive reaction to anger in Turkish content and its negative reception in English content underscores the complex role that emotions play in brand engagement across cultures. This suggests that Turkish-speaking audience may be more responsive or tolerant to confrontation or passionate expression on social media, while English-speaking audience may prefer more positive or neutral emotional tones. The universal appeal of happiness, even at different levels of significance, points to its potential as a cross-cultural tool for enhancing consumer engagement, albeit with adjustments to match each audience's unique cultural context. The higher effectiveness of vividness elements such as emojis and images in engaging English-speaking audiences further suggests a preference for visually rich and emotionally evocative content in these markets. This finding is compatible with Schultz (2017) and Antoniadis et al. (2019). Regarding the Turkish-speaking market, such elements, while still valued, do not hold as much weight, potentially indicating a variance in visual communication's role and emotional content across cultures.
The higher importance of daytime posting in the English-speaking market compared to the Turkish-speaking market emphasized the role of content timing in maximizing engagement, possibly reflecting differences in daily routines, work hours and social media usage patterns between cultures. For the English-speaking markets, contradictory results were presented regarding the effects of weekday messaging and day time messaging by Schultz (2017) and Antoniadis et al. (2019). The timing of posts, evidenced by the differing impacts of daytime posting across markets, illustrates the necessity of understanding the daily lives, routines and peak social media usage times of different cultural groups. This practical aspect of content strategy, informed by cultural norms and lifestyle patterns, shows the necessity for marketers to adopt a localized approach in their global social media campaigns, ensuring that content is not only contextually cultural-relevant but also timely.
Interactive elements like hashtag and question usage exhibited a subtle preference in English-speaking markets, suggesting a culture that values dialogue and direct interaction within social media spaces, where the hypothesis of higher levels of interactivity resulting in more interactions were partially accepted by De Vries et al. (2012) and later by Schultz (2017). This is aligned with individualistic tendencies that encourage self-expression and public discourse. In contrast, the Turkish-speaking market's engagement patterns reflected a balanced view toward interactivity, possibly prioritizing content's emotional or informational resonance over its interactive features. Moreover, the relative importance of vividness in the English-speaking context points to the necessity of incorporating culture-specific insights into content creation to enhance engagement.
Informativeness in messages was shown to be effective on post popularity by Antoniadis et al. (2019), while no significant relation was found previously by De Vries et al. (2012). Majority of the previous work discussed that entertaining content had no effect or negative impact on post interactions. The significantly higher importance of information in the English-speaking market compared to the Turkish-speaking market suggested that English-speaking consumers may engage more deeply with content that enhances their knowledge or provides practical insights. Conversely, the entertainment variable's varied impact across cultures highlights the challenge of creating content that universally appeals across diverse audiences, suggesting the need for a balanced content strategy that integrates both informative and entertaining elements tailored to the cultural nuances of each target audience.
The comparative analysis of consumer engagement across English- and Turkish-speaking markets showed the critical need for culturally sensitive social media marketing strategies. Understanding the cultural underpinnings of consumer behavior, emotional engagement, content preferences and the optimal timing of interactions can guide global brands in creating more effective, engaging and resonant social media content locally.
5.2 Theoretical and methodological implications
In addition to its primary objectives and key discoveries, this research addressed several previously underexplored issues. First, it demonstrated the utility of random subspace regression using bootstrap for more accurate estimation of feature coefficients, confidence intervals and p-values, effectively assessing their significance and importance in social media analysis across different languages. It addressed gaps in previous methodologies by offering a more precise evaluation of variable effects, validated through 1,000 iterations that revealed higher explained variance and lower error rates than traditional models. This approach's success highlights its efficiency and reliability, especially beneficial for handling complex datasets that do not meet conventional statistical models' normal distribution, linear relationships or homoscedasticity assumptions. The bootstrap method's flexibility and robustness make it valuable for high-dimensional data especially for social media research, by enabling insights from complex datasets with increased confidence.
The study also theoretically contributes to the fields of digital marketing, branding and communication disciplines. As suggested by Lin et al. (2017), cultural perspectives are believed to mediate the impact of content features on consumers' appeal to brands' social media messages. Consumer factors such as social influence, perceived social risk and personal traits are among the factors that influence social media consumer engagement (Barger et al., 2016). Additionally, content features and cultural perspectives of the society in which consumers live can also impact consumer engagement. Former studies focused on comparing USA and Asian countries as China, Japan or South Korea to investigate the cross-cultural consumer behavior, while research on transition economies could offer overarching insights that researchers and social media managers might apply to a wider spectrum of markets (Cummins et al., 2014). This research incorporates the consumer dynamics from a Turkish-speaking market, an emerging economy, to compare the dynamics with the English-speaking ones.
The study's findings illuminate the significance of cultural context in shaping the effectiveness of various content features – such as vividness, practicality, interactivity, information and entertainment – in brand posts. Additionally, the examination of emotional tones in brand messages across English and Turkish datasets and their differential impact on brand post engagement offers certain insights into the role of emotion in marketing and brand communication. This underscores a critical need for marketing theories to incorporate cross-cultural insights, moving beyond monolithic strategies to embrace an approach that accounts for cultural diversity. Traditional models, which may not fully account for cultural differences in perception and engagement, could be refined to better predict consumer responses in a globalized marketplace.
5.3 Managerial implications
This study investigated the dynamics of brand messaging on social media in English- and Turkish-speaking markets. The aim was to understand the cultural diversity that shapes the perception of content elements in different markets, and how this diversity impacts the popularity of brand posts. Results showed that understanding the cultural variety that influences how different markets perceive content features can significantly enhance brand post engagement. Naturally, understanding the dynamics across different markets, social media platforms and time also require a data-driven approach that works with confidence. Due to the increased accessibility of cost-effective computing power, sampling methods such as the one illustrated and the subsequent analysis of the data help improve our understanding of the effects of content qualities in situations where conventional methods do not meet specific assumptions. By aligning their social media content strategy with market characteristics, brands can enhance consumer engagement through more likes, shares and comments. The increased level of engagement will likely have consequences on brand loyalty, perceived brand quality, attitude toward the product, purchase intentions and finally sales (Barger and Labrecque, 2013; Barger et al., 2016; Cummins et al., 2014).
Findings showed that the preference for daytime messages among English-speaking consumers and nighttime messages among Turkish-speaking consumers suggests the need for dynamic content scheduling strategies that align with the peak activity times of the target audience. This also implies leveraging analytics to understand not just what content resonates but when it is most likely to be seen and engaged with. The variable importance of information and entertainment across both datasets also showed their role as key engagement drivers. For instance, in relatively more collectivist cultures represented by the Turkish data, marketers can emphasize emotional appeals and community-centric messages. In contrast, in individualistic cultures like those represented by the English data, brands can focus more on providing informative and entertaining content that appeals to personal interests and preferences. The significance of interactivity, especially regarding English-speaking consumers, in enhancing brand post popularity underscores the potential of using interactive content as a tool for community building. Brand managers can foster a sense of community among their audience by encouraging user-generated content though hashtags, mentions or questions to boost consumer engagement.
The study's findings on the differential impact of emotional tones across cultural contexts suggest that brands should carefully consider the emotional underpinning of their brand messages. Integrating storytelling to posts can elevate brand posts from mere conveyance of information to engaging narratives that captivate the audience. Brands can weave emotions into stories that reflect the brand's values, customer success stories or how the brand's products or services fit into the larger context of consumers' lives. This approach would not only inform but also entertain and connect emotionally with the audience, enhancing the overall impact of the content. Beyond just aiming to evoke happiness or sadness, it might also be beneficial to explore a broader range of emotions that could resonate with specific cultural or demographic groups analyzing data with the approaches presented. For instance, content that inspires awe or curiosity might be particularly effective in educational or technological fields, while content that evokes nostalgia or sentimentality could be more impactful for brands with a long history or those marketing products related to personal memories.
Marketers can leverage the significant value of social media to effectively utilize and control the available data in order to address decisions related to social media that are interconnected (Schultz, 2016, 2017). For example, this study's novel use of observable data in both English- and Turkish-speaking markets enables brands to employ suitable strategies and make informed decisions in these markets. Brand managers can enhance their social media interaction strategy by utilizing data from various markets and social media platforms.
6. Conclusion and future research directions
This study aimed to investigate the factors that contributed to the popularity of brand posts on platform X in English- and Turkish-speaking markets. The focus was on understanding the role of content characteristics in driving the brand post popularity. While this study provides valuable insights into the cross-cultural analysis of brand post popularity, several limitations should be acknowledged. Firstly, its findings may not be broadly applicable to different cultural contexts due to the analysis being based solely on English and Turkish data. Future research should include a more diverse set of languages to capture cross-cultural variations, especially in eastern and western cultures.
Secondly, the dataset used in the analysis may have a specific time range, potentially not reflecting the most up-to-date trends and dynamics in brand post popularity. To address this limitation, future research should consider updating the data with a more current time frame to gain a more accurate understanding of how brand post interactions change over time. Moreover, investigating the impact of time on brand post popularity, including temporal dynamics, seasonal effects, trending topics or specific events, would provide valuable insights.
Lastly, this study analyzed only a subset of features and emotions related to brand posts. To obtain a more comprehensive understanding of the factors that influence post popularity, future research should broaden its scope. This could involve incorporating additional features, such as sentiment analysis of accompanying visuals, and exploring the values conveyed through brand posts. Expanding the range of analyzed features would offer a more detailed and nuanced perspective on the determinants of brand post popularity.
In conclusion, although this study offers valuable insights into the cross-cultural examination of consumer brand post engagement, future research should strive to overcome the limitations by incorporating a broader array of languages, platforms, employing more up-to-date data, broadening the scope of analyzed features and emotions and considering the temporal dimension in brand post interactions. By addressing these constraints, researchers and brands can advance our comprehension of the complex dynamics involved in consumer engagement across diverse cultural markets.
Credits
Figures and Tables are derived from the author's own work presented in the following thesis: Tanaltay (2023). Cross cultural analysis of emotions on social media branding communication with evidence from big data (Doctoral Dissertation). Sabanci University, Turkey.
Figures
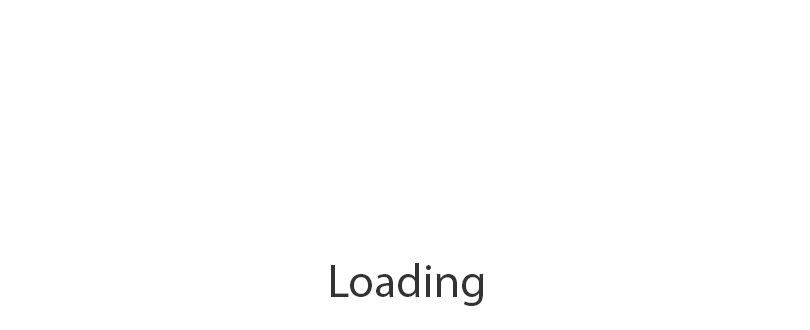
Figure 1.
Conceptual framework (Tanaltay, 2023), inspired by Antoniadis et al. (2019), De Vries et al. (2012) and Schultz (2017)
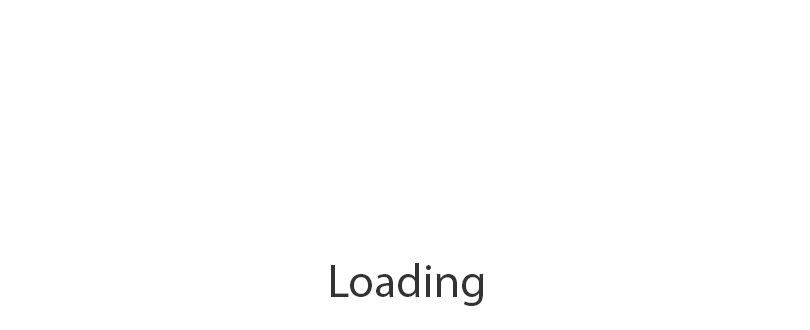
Figure 2.
Research design (Tanaltay, 2023)
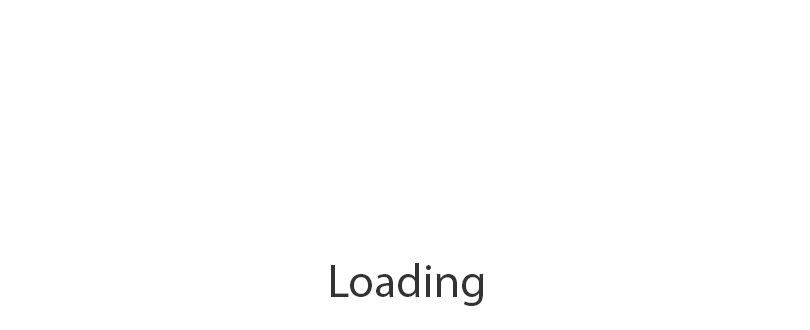
Figure 3.
Average importance scores by feature group (Tanaltay, 2023) – sorted in decreasing order by English importance scores
Company | Industry | Company | Industry | Company | Industry |
---|---|---|---|---|---|
Gillette | FMCG | McDonald's | Fast Food | Microsoft | Technology |
Coca Cola | FMCG | Alldays | FMCG | HP | Technology |
L'Oreal | FMCG | Pepsi | FMCG | Huawei | Technology |
Algida/Walls | FMCG | Toyota | Automotive | Allianz | Insurance |
Burger King | Fast Food | BMW | Automotive | Axa | Insurance |
KFC | Fast Food | H&M | Apparel | HSBC | Banking |
Vodafone | Technology | Watsons | Retail | Netflix | Technology |
Samsung | Technology | Flormar | FMCG | UPS | Logistics |
Hyundai | Automotive | Oriflame | FMCG | DHL | Logistics |
Levi's | Apparel | Carrefour | Retail | ||
Marks&Spen. | Apparel | Yves Rocher | FMCG | ||
Siemens | Technology | Sony | Technology |
Turkish | English | |||||
---|---|---|---|---|---|---|
Industry | Posts | Followers | Interactions | Posts | Followers | Interactions |
Logistics | 1,700 | 61,141 | 52,707 | 22,785 | 299,371 | 846,139 |
Personal Care | 2,269 | 76,621 | 127,525 | 8,564 | 421,327 | 1,394,644 |
Food Service | 3,286 | 39,342 | 136,144 | 4,785 | 8,015,842 | 19,113,026 |
Food & Beverage | 856 | 135,096 | 232,423 | 5,676 | 6,427,611 | 2,782,856 |
Financial Services | 3,364 | 49,412 | 287,831 | 5,348 | 329,809 | 433,440 |
Retail | 9,678 | 426,035 | 494,603 | 14,763 | 9,772,636 | 2,401,469 |
Automotive | 3,992 | 492,354 | 571,561 | 14,323 | 3,456,692 | 13,847,786 |
Technology | 12,680 | 3,656,490 | 11,227,930 | 25,726 | 25,569,968 | 16,583,574 |
Features | Description | Type | Value |
---|---|---|---|
Vividness | |||
Emojis | Number of emojis in a post. | Discrete, numerical | ≥0 |
Images | Number of images in a post. | Discrete, numerical | ≥0 |
Videos | Number of videos in a post. | Discrete, numerical | ≥0 |
Interactivity | |||
Hashtags | Number of hashtags in a post. | Discrete, numerical | ≥0 |
Links | Number of links in a post. | Discrete, numerical | ≥0 |
Mentions | Number of user mentions in a post. | Discrete, numerical | ≥0 |
Poll | Post is a poll. | Binary | 1: if poll, 0: otherwise |
Question | Post is a question. | Binary | 1: if question, 0: otherwise |
Practicality | |||
Daytime | Post is submitted in daytime. | Binary | 1: if daytime, 0: otherwise |
Weekday | Post is submitted on weekdays. | Binary | 1: if weekdays, 0: otherwise |
Position | Duration at top position in minutes. | Discrete, numerical | ≥0 |
Text length | Number of characters in post message. | Discrete, numerical | ≥0 |
Holiday | If the post is about a holiday. | Binary | 1: if about holiday, 0: otherwise |
Emotional appeal | |||
Happy | Post stimulates happiness. | Binary | 1: if happy, 0: otherwise |
Surprise | Post stimulates surprise. | Binary | 1: if surprise, 0: otherwise |
Sad | Post stimulates sadness. | Binary | 1: if sad, 0: otherwise |
Anger | Post stimulates anger. | Binary | 1: if anger, 0: otherwise |
Disgust | Post stimulates disgust. | Binary | 1: if disgust, 0: otherwise |
Fear | Post stimulates fear. | Binary | 1: if fear, 0: otherwise |
Neutral | No emotions are attached to the post. | Base variable | |
Information | Post is about a product. | Binary | 1: if about product, 0:otherwise |
Entertainment | Post is about sports, TV, music, events. | Binary | 1: if entertaining, 0:otherwise |
Industry (Control) | |||
Logistics | Brand in Logistics industry. | Binary | 1: if from Logistics, 0:otherwise |
Food Service | Brand in Food Service industry. | Binary | 1: if Food Service, 0:otherwise |
Personal Care | Brand in Personal Care industry. | Binary | 1: if Personal Care, 0:otherwise |
Retail | Brand in Retail industry. | Binary | 1: if Retail, 0:otherwise |
Financial Services | Brand in Finance industry. | Binary | 1: if Financial Ser., 0:otherwise |
FMCG | Brand in FMCG industry. | Binary | 1: if FMCG, 0:otherwise |
Automotive | Brand in Automotive industry. | Binary | 1: if Automotive, 0:otherwise |
Technology | Brand in Technology industry. | Base variable | |
Followers (Control) | Number of followers. | Discrete, numerical | ≥0 |
Appendix 1. Fortune 500 brands selected as sample
Appendix 2. Total number of posts, followers and interactions by industry
Appendix 3. Quantitative representation of features by feature categories
Appendix 4. Mathematical description of regression models evaluated
In order to discover the most impacting features and their direction of effect, also in line with previous research, the linear relation in Equation 1 is used to model brand post popularity for English and Turkish languages:
Then, the linear relation described in Equation 1 is used to fit OLS, Poisson and NB regression models in order to find the best distribution to explain the variability of brand post popularity measured as yi. OLS, Poisson and NB versions of the regression models are described in Equations 2, 3 and 4, respectively.
OLS regression:
Poisson regression:
NB regression:
Regarding PR variance is constant and equal to the mean λ, where in NB regression accounts for an extra dispersion parameter Γ to model the over-dispersed count data. Regarding both models a closed form solution to find the best β's doesn't exist, thus, maximum likelihood estimation (MLE) is used to find the coefficient estimates that maximize the likelihood of the observed data given the model.
Subsequently, the linear relationship outlined in Equation 1 is employed to apply and evaluate OLS, PR and NB regression models. This is done with the aim of determining the most suitable distribution for elucidating the variation in brand post popularity, quantified as yi given independent variables. Regarding the OLS regression model, dependent variable is natural log transformed. All models were fit using MLE, which determines the coefficients of variables by maximizing the likelihood of observing the given data under the model. The models' goodness-of-fit is assessed using the Akaike information criterion (AIC), which gauges the comparative performance of statistical models with respect to a given dataset (Stoica and Selen, 2004).
In this context, lower AIC scores are considered more advantageous when it comes to model selection. The results of the fitting procedure are shown below:
Assessment of regression model
The OLS regression model, based on log-transformed total interaction counts, exhibits the lowest AIC scores for both English (39.56) and Turkish (13.20) datasets showing that it is the closest match, as illustrated by the blue and red lines in the figure. For both languages, the OLS model demonstrates a satisfactory ability to account for the skewness of distributions, particularly in the right tails. Consequently, the OLS regression model was chosen for further examination of the impact of variables on consumer interactions using random subspaces on bootstrap samples.
Appendix 5. Estimation of parameters using random subspace regression with bootstrap
Bootstrap is a resampling technique used to estimate the distribution of a test statistic or parameter by repeatedly sampling the data with replacement. It involves generating multiple samples and calculating the statistic of interest for each sample, allowing for the estimation of sampling distributions, confidence intervals and standard errors (Efron and Tibshirani, 1994). In the context of linear regression, the estimate is obtained as the average of coefficients derived from all bootstrap models, while the 0.025 and 0.975 quantiles define the 0.95 confidence interval for this estimate. If this interval contains zero, it indicates that the null hypothesis, which suggests that the coefficient's value is zero, cannot be rejected. This approach is particularly useful when statistical assumptions are violated or dealing with large datasets (Horowitz, 2019). Ensemble techniques like bagging, random subspaces and random patches leverage bootstrapping by building multiple models with full sets of variables on B bootstrap samples, randomly selecting subsets of features B times and fitting them to the full dataset, or both, respectively. Moreover, random subspace regression, a specific application, employs random feature selection on bootstrapped samples to streamline analysis, especially beneficial for extensive feature sets or large datasets and when the underlying distribution of the variable in concern is nonnormal and do not meet the assumptions of OLS (Boot and Nibbering, 2019).
Concerning the dataset of brands, the interactions exhibit a skewed distribution, and the relationship between independent and dependent variables is nonlinear. Additionally, despite the mean variance inflation factor (VIF) for English and Turkish being relatively low at 2.77 and 2.80, respectively, there is a notable degree of multicollinearity. While most individual VIF scores are below 10, natural correlations exist among independent variables, such as the co-occurrence of images or videos with links and the interplay between links, mentions, hashtags and emojis in affecting post length. These factors raise concerns about the potential presence of heteroscedasticity in the residuals of an OLS model, which can impact the reliability of coefficient estimates.
To address these issues and enhance the robustness of inference, a solution inspired by previous work by Diaz-Diaz et al. (2005) and later by Wichitaksorn et al. (2023a) random subspace regression using the bootstrap method was employed. It was adapted to estimate coefficients, their associated confidence intervals, p-values and an importance score for each coefficient concerning the given features and conceptual model. Resampling with multiple iterations was employed on both English and Turkish datasets to create OLS regression models based on randomly selected features and observations. In this process, we have input data denoted as D, a set of features labeled as P with each feature indexed as p, a sample size represented by n, the number of bootstrap iterations as b and the maximum number of randomly chosen features set at j. In each iteration, we randomly drew a sample of n observations, referred as Dboot from the dataset D. The remaining observations, designated as Doob, were considered out-of-bag and reserved for calculating the importance score. Within each iteration, we selected a random sample of features, ranging from a minimum of 1 to a maximum of j, and employed these features to fit an OLS regression model on the dataset Dboot. This resulted in each regression model for the ith bootstrap iteration having a different number of randomly chosen predictors. As the number of parameters in all bootstrap models were different, the feature importance score was calculated based on explained variance of the model using the adjusted R2, which is resistant to increasing number of variables in the regression models penalizing the score down when the number of parameters increase. At the conclusion of each iteration, the estimate for each selected coefficient is stored in the vector
Once all bootstrap iterations were completed, we calculated the coefficient estimate for each feature in P as the average of the values in
The bootstrap regression models were fit using the StatsModels library, and bootstrap resampling was implemented in Python development environment. To determine the minimum number of bootstrap iterations required to effectively identify the optimal set of features explaining the variance, the bootstrap procedure was applied iteratively with 50, 100, 500, 1,000 and 5,000 iterations. For each iteration, the sample size n comprised 10,000 observations, and a maximum of ten features were randomly selected from the complete set of available features. The RMSE was computed for each bootstrap cycle as the number of iterations increased, as illustrated in Figure “Performance of different number of bootstrap iterations.” Within each cycle, features with the highest importance scores and p-values less than the predefined significance level (alpha) of 0.01 were chosen and subjected to cross-validated RMSE evaluation using the OLS model on the out-of-bag data. Additionally, the cross-validated RMSE of the full model, which included all features, was calculated on the entire dataset for comparison (see the blue line in Figure “Performance of different number of bootstrap iterations”). Notably, the bootstrap approach consistently yielded lower RMSE values for both languages when contrasted with the full model. The lowest RMSE was achieved when the bootstrap cycle was run for 1,000 iterations, a marked improvement over feature subset selection via exhaustive search, which would require exploring 229 alternative combinations, considering that 29 features were under consideration for the linear model. In summary, using bootstrap resampling for feature selection with a focus on minimizing RMSE over 1,000 iterations is a highly efficient, computationally feasible and effective method for identifying the most relevant features for a linear model, especially when compared to the exhaustive search method. This approach not only aids in building a more accurate and generalizable model but also offers insights into feature importance, contributing to a deeper understanding of the underlying data structure.
Performance of different number of bootstrap iteration
Red line: cross-validated RMSE of selected model after bootstrap iterations; blue line: cross-validated RMSE of the full model.
Appendix 6. Random subspace bootstrap experiment coefficient estimates
Each category is sorted according to importance score in decreasing order:
References
Antoniadis, I., Paltsoglou, S. and Patoulidis, V. (2019), “Post popularity and reactions in retail brand pages on Facebook”, International Journal of Retail & Distribution Management, Vol. 47 No. 9, pp. 957-973. doi: 10.1108/IJRDM-09-2018-0195.
Banerjee, S. and Chua, A.Y.K. (2019), “Identifying the antecedents of posts' popularity on Facebook Fan Pages”, Journal of Brand Management, Vol. 26, pp. 621-633.
Barger, V. and Labrecque, L. (2013), “An integrated marketing communications perspective on social media metrics”, International Journal of Integrated Marketing Communications, Spring, Vol. 5 No. 1, pp. 64-76.
Barger, V., Peltier, J.W. and Schultz, D.E. (2016), “Social media and consumer engagement: a review and research agenda”, Journal of Research in Interactive Marketing, Vol. 10 No. 4, pp. 268-287. doi: 10.1108/JRIM-06-2016-0065.
Berry, W.D. (1993), Understanding Regression Assumptions, Sage, Newbury Park.
Boot, T. and Nibbering, D. (2019). “Forecasting using random subspace methods”, Journal of Econometrics, Vol. 209 No. 2, pp. 391-406.
Crosby, L. and Johnson, S. (2007), “Experience required”, Marketing Management, Vol. 16 No. 4, pp. 21-28.
Cummins, S.W., Peltier, J.A., Schibrowsky, J. and Nill, A. (2014), “Consumer behavior in the online context”, Journal of Research in Interactive Marketing, Vol. 8 No. 3, pp. 169-202. doi: 10.1108/JRIM-04-2013-0019.
De Vries, L., Gensler, S. and Leeflang, P.S.H. (2012), “Popularity of brand posts on brand fan pages: an investigation of the effects of social media marketing”, Journal of Interactive Marketing, Vol. 26 No. 2, pp. 83-91.
Delia, E.B. and Armstrong, C.G. (2015), “#Sponsoring the #FrenchOpen: an examination of social media buzz and sentiment”, Journal of Sport Management, Vol. 26, pp. 184-199. doi: 10.1123/JSM.2013-0257.
Diaz-Diaz, N., Aguilar-Ruiz, J.S., Nepomuceno, J.A. and Garcia, J. (2005). “Feature selection based on bootstrapping”, Paper presented at the 2005 ICSC Congress on Computational Intelligence Methods and Applications.
Edelman, D. (2010), “Branding in the digital age”, Harvard Business Review, Vol. 88 No. 12, pp. 62-69.
Efron, B. and Tibshirani, R.J. (1994), An Introduction to the Bootstrap, CRC press, Chapman and Hall, New York.
Ekinci, Y., Yoon, T.H. and Oppewal, H. (2004), “An examination of the brand relationship quality scale in the evaluation of restaurant brands”, Advances in Hospitality and Leisure, Vol. 1, pp. 189-197. doi: 10.1016/S1745-3542(04)01012-4.
Geurin, A.N. and Burch, L.M. (2017), “User-generated branding via social media: an examination of six running brands”, Sport Management Review, Vol. 20 No. 3, pp. 273-284.
Hofstede, G. (2011), “Dimensionalizing cultures: the Hofstede model in context”, Online Readings in Psychology and Culture, Vol. 2 No. 1, p. 8. Retrieved from: http://scholarworks.gvsu.edu/orpc/vol2/iss1/8
Hofstede, G., Hofstede, G.J. and Minkov, M. (2010), Cultures and Organizations: Software of the Mind, McGraw-Hill, New York.
Horowitz, J.L. (2019). “Bootstrap methods in econometrics”, Annual Review of Economics, Vol. 11, pp. 193-224.
Hudson, S., Roth, M.S., Madden, T.J. and Hudson, R. (2015), “The effects of social media on emotions, brand relationship quality, and word of mouth: an empirical study of music festival attendees”, Tourism Management, Vol. 47, pp. 68-76. doi: 10.1016/j.tourman.2014.09.001.
Izogo, E.E. and Mpinganjira, M. (2020), “Behavioral consequences of customer inspiration: the role of social media inspirational content and cultural orientation”, Journal of Research in Interactive Marketing, Vol. 14 No. 4, pp. 431-459. doi: 10.1108/JRIM-09-2019-0145.
Karpinska-Krakowiak, M. and Modlinski, A. (2020), “Popularity of branded content in social media”, Journal of Computer Information Systems, Vol. 60 No. 4, pp. 309-315.
Laverie, D.A., Kleine, R.E. and Kleine, S.S. (1993), “Linking emotions and values in consumption experiences: an exploratory study”, Advances in Consumer Research, Association for Consumer Research, Vol. 20, pp. 70-75.
Lee, C. and Kahle, L. (2016), “The linguistics of social media: communication of emotions and values in sport”, Sport Marketing Quarterly, Vol. 25 No. 4, pp. 201-211.
Lin, H.-C., Swarna, H. and Bruning, P.F. (2017), “Taking a global view on brand post popularity: six social media brand post practices for global markets”, Business Horizons, Vol. 60 No. 5, pp. 621-633.
Mazloom, M., Rietveld, R., Rudinac, S., Worring, M. and Van Dolen, W. (2016), “Multimodal popularity prediction of brand-related social media posts”, Paper presented at the Proceedings of the 24th ACM International Conference on Multimedia, pp. 197-201. doi: 10.1145/2964284.2967210.
Pinto, M.B. and Yagnik, A. (2017), “Fit for life: a content analysis of fitness tracker brands use of Facebook in social media marketing”, Journal of Brand Management, Vol. 24, pp. 49-67.
Sabate, F., Berbegal-Mirabent, J., Caabate, A. and Lebherz, P.R. (2014), “Factors influencing popularity of branded content in Facebook fan pages”, European Management Journal, Vol. 32 No. 6, pp. 1001-1011.
Schultz, C.D. (2016), “Driving likes, comments, and shares on social networking sites: how post characteristics affect brand interactions in apparel retailing”, Paper presented at the Proceedings of the 18th Annual International Conference on Electronic Commerce: E-Commerce in Smart connected World, pp. 1-9. doi: 10.1145/2971603.2971612.
Schultz, C.D. (2017), “Proposing to your fans: which brand post characteristics drive consumer engagement activities on social media brand pages?”, Electronic Commerce Research and Applications, Vol. 26, pp. 23-34.
Schultz, D.E. and Peltier, J.W. (2013), “Social media's slippery slope: challenges, opportunities and future research directions”, Journal of Research in Interactive Marketing, Vol. 7 No. 2, pp. 86-99. doi: 10.1108/JRIM-12-2012-0054.
Stoica, P. and Selen, Y. (2004). “Model-order selection: a review of information criterion rules”, IEEE Signal Processing Magazine, Vol. 21 No. 4, pp. 36-47.
Swani, K., Milne, G. and Brown, B.P. (2013), “Spreading the word through likes on Facebook: evaluating the message strategy effectiveness of Fortune 500 companies”, Journal of Research in Interactive Marketing, Vol. 7 No. 4, pp. 269-294.
Swani, K. and Milne, G.R. (2017), “Evaluating Facebook brand content popularity for service versus goods offerings”, Journal of Business Research, Vol. 79, pp. 123-133.
Tanaltay, A. (2023), Cross Cultural Analysis of Emotions on Social Media Branding Communication with Evidence from Big Data, Sabanci University, Turkey.
Vander Schee, B.A., Peltier, J. and Dahl, A.J. (2020), “Antecedent consumer factors, consequential branding outcomes and measures of online consumer engagement: current research and future directions”, Journal of Research in Interactive Marketing, Vol. 14 No. 2, pp. 239-268. doi: 10.1108/JRIM-01-2020-0010.
Wichitaksorn, N., Kang, Y. and Zhang, F. (2023a), “Random feature selection using random subspace logistic regression”, Expert Systems with Applications, Vol. 217, p. 119535. doi: 10.1016/j.eswa.2023.119535.
Yu, C.-E. and Sun, R. (2019), “The role of Instagram in the UNESCO's creative city of gastronomy: a case study of Macau”, Tourism Management, Vol. 75, pp. 257-268.
Zadeh, A.H. and Sharda, R. (2014), “Modeling brand post popularity dynamics in online social networks”, Decision Support Systems, Vol. 65, pp. 59-68.
Further Reading
Wichitaksorn, N., Kang, Y. and Zhang, F. (2023b). “Random feature selection using random subspace logistic regression”, Expert Systems with Applications, Vol. 217, p. 119535.
Acknowledgements
This manuscript disseminates partial results from the thesis studies of Altug Tanaltay, an A.B.D. Ph.D. Graduate at the Sabanci Business School titled “Cross Cultural Analysis of Emotions on Social Media Branding Communication with Evidence from Big Data”, 2023, under co-supervision of Prof. Nihat Kasap and Prof. Selcen Ozturkcan.