Abstract
Purpose
“Internet of things” is a broad term used to describe network connectivity to physical objects. Called connectable or smart objects, they are embedded in electronic circuits and software that make them capable of detecting, collecting and transmitting data and information. This paper aims to examine the influence of the attributes of “internet of things” products in the functional and emotional experience of purchase intention.
Design/methodology/approach
The study used a model adapted from Yaping et al. (2014), with adjustment for the constructs “emotional experience”, “functional experience” and “purchase intention”. The survey consisted of a sample of 747 valid questionnaires regarding users of “internet of things” products, through a structured questionnaire with 36 assertions, which were answered based on the Likert scale. The quantitative research approach followed an exploratory descriptive phase followed by the application of structural equation modeling.
Findings
Results validated most of the relationships of the model, with high levels of significance. In addition, there was a greater influence of emotional experience than functional on purchase intention for the selected sample, which mainly consisted of young people.
Originality/value
In short, the study confirmed the statistical significance of the structural paths, indicating that the proposed model is consistent, and with an appropriate adjustment can be applied in future research.
Keywords
Citation
Pinochet, L.H.C., Lopes, E.L., Srulzon, C.H.F. and Onusic, L.M. (2018), "The influence of the attributes of “Internet of Things” products on functional and emotional experiences of purchase intention", Innovation & Management Review, Vol. 15 No. 3, pp. 303-320. https://doi.org/10.1108/INMR-05-2018-0028
Publisher
:Emerald Publishing Limited
Copyright © 2018, Luis Hernan Contreras Pinochet, Evandro Luiz Lopes, Caio Henrique Fernandes Srulzon and Luciana Massaro Onusic.
License
Published in Innovation & Management Review. Published by Emerald Publishing Limited. This article is published under the Creative Commons Attribution (CC BY 4.0) licence. Anyone may reproduce, distribute, translate and create derivative works of this article (for both commercial and non-commercial purposes), subject to full attribution to the original publication and authors. The full terms of this licence may be seen at http://creativecommons.org/licences/by/4.0/legalcode
1. Introduction
Technological revolution is driven by information technologies and modes of communication in our contemporary society. As a result, the internet has grown fast and currently has 2.5 billion active users, most of them young people (Younes et al., 2016).
If we examine the use of information and communication technology (ICT), a good part of what we do through our gadgets (mobile devices of various segments: smartphones, tablets, PCs and smartwatches, among others) has services that are connected to cloud computing and “internet of things” (IoT) systems (Hancock and Hancock, 2016).
IoT is a broad term that describes network connectivity to physical objects. These are called connectable or intelligent objects, and are embedded in electronic circuits and software that enable them to detect, collect and transmit data and information.
The term was originated in the work developed in the Auto-ID Labs at Massachusetts Institute of Technology (MIT), on network radio-frequency identification (RFID; Wortmann and Flüchter, 2015). It was first introduced to society by Ashton (2009), in a presentation about the RFID device and supply chain. Ashton was probably the first researcher to use this term, describing a vision of sensors, devices and people or internet connectable citizens (Boulos and Al-Shorbaji, 2014).
Among several studies, Yaping et al.’s (2014) model, published in the article “Influence of characteristics of the IoT on consumer purchase intention”, in the international academic journal Social Behavior and Personality, stands out in the literature by measuring the effect of the attributes of IoT products on the emotional and functional experiences, and consequently on purchase intention. In the proposed model, the authors highlight six features of these products: connectivity, interactivity, sense of presence, intelligence, convenience and security.
Each attribute has a direct relationship with consumers’ emotional and functional experiences, and how each one of them affects the intention to buy the product. The functional experience of the product–customer relationship stems, to a large extent, from the satisfaction with the utility perceived in IoT technology. However, there is a tendency for companies to strengthen the bonds of emotional experience. We chose to analyze the IoT to deepen the understanding of the relationship of this concept with the purchase intention of these products.
Harvard Business Review highlighted, in the article “How smart, connected products are transforming competition” (Porter and Heppelmann, 2014), that a range of innovations in technologies is turning them into intelligent objects with economic attractiveness (reduction of electricity consumption, higher efficiency of sensors and batteries, low-cost products and wireless network, among others).
IoT has strongly influenced changes in businesses and in the whole society. Its expansion will affect consumers, companies, governments and hospitals, among other users (Said and Masud, 2013). Rivera and Meulen (2014) estimated that 2.9 billion of IoT devices would be used in the consumer sector in 2015, reaching 13 billion by 2020.
Although the potential benefits that IoT can bring to companies, by allowing a better adjustment to changes in the environment, such as agility in decision-making, few studies focus on businesses, through the proposition of an adoption strategy or consumption analysis of IoT devices (Bąk et al., 2015; Galegale et al., 2016).
The purpose of this article is to identify the main attributes of IoT products that affect the emotional and functional experiences of purchase intention. Thus, it contributes to map the scope and market opportunities for IoT products; there is a growing demand, which tends to cross ever deeper layers of the market, starting with the young – currently the most connected age group in society.
This article is structured as follows: introduction; theoretical background, which provides the conceptual basis of the theme; building of the theoretical model, method, data analysis and conclusions.
2. Literature review
This section presents the theoretical concepts and studies that supported the research. To facilitate understanding, we divided the literature review in IoT and its potential applications, and consumer’s behavior toward these technologies.
2.1 Internet of things
Three capacities of the IoT have significant ramifications at the individual and societal levels: data collection, capacity of remote control and communication between objects (Hancock and Hancock, 2016).
The evolution of internet has brought about an intelligent exchange of information between objects, enabling the creation of a new range of products and services in the real and virtual worlds (Sánchez-Alcón et al., 2015). Solutions are extending to all areas of everyday life, including, the intelligent industry (intelligent manufacturing systems – industry 4.0), smart homes and intelligent transportation (solutions that include fleet tracking, mobile ticketing, etc.; Wortmann and Flüchter, 2015).
For Atzori et al. (2010), the central idea of IoT is the invasive presence of several objects with single addresses, which can interact and achieve common goals. Through the internet, several resources can be shared with each other (Chen et al., 2013).
The more objects are added to the IoT, the more opportunities for communication machine-to-machine will arise, thus extending the possibilities of automation of daily tasks, personalization of profiles and integration of other sides of everyday life (Sánchez-Alcón et al., 2015; Hancock and Hancock, 2016).
According to Ashton (2009), society lives in a paradigm in which users no longer control the time, duration and place for computer use; now processing is in real time and scattered in the environment. Therefore, it is necessary to understand the main potential applications of IoT, as presented in Table I.
Hence, it is imperative to identify how consumers observe the changes regarding the different applications presented in Table I as they modify the way people relate to the world, and directly affect personal and professional lives. IoT can bring opportunities that must be noticed, but, accordingly, the degree of risk of devices that are connected to the network can increase. Thus, it is necessary to know what drives consumers to make use of these technologies.
2.2 Consumer behavior regarding internet of things technologies
In a society where people can choose the electronic devices they carry most of the time, IoT objects can bring many advantages. Competition between these products in the market can offer several ways to differentiate services and add value, which benefits consumers.
The technological acceptance model, one of the most popular models that explain the acceptability of these new technologies, suggests that the use of these technologies can be explained by the degree of user’s motivation, which is directly affected by incentives, such as design and performance (Roman et al., 2015).
The attributes of the IoT products reach consumers’ feelings and understanding, also affecting their objective cognition (functional experience) and subjective cognition (emotional experience), providing them the conditions to select the most appropriate technology.
3. Theoretical model building and hypotheses
On the basis of the features of IoT products developed by Yaping et al. (2014), who analyze consumers’ purchase intention, we built the theoretical model and the hypotheses related to the six dimensions: connectivity, interactivity, sense of presence, intelligence, convenience and security, as well as the relationship of these attributes with functional experience (objective cognition) and emotional experience (subjective cognition), as follows:
3.1 Connectivity
IoT is the latest evolution of intelligent objects that connect the physical world to collected information and data. These smart objects have many attributes and can be connected to a device or network. They also have the ability to manage, monitor and track objects to provide information such as instructions, tutorials and controls to the user. Originally, internet’s intention was to carry information to anyone, anywhere, anytime, but currently “anyone” has been replaced by “anything” (Eisenberg and Fullerton, 2012). Social networks are defined as a set of independent users that communicate through a given network, interacting with each other, joining objectives. Social networks are dynamic and allow the creation of relationships according to their needs (Yaping et al., 2014). Thus, we propose the following hypotheses:
The connectivity of “Internet of Things” will have a positive effect on consumers’ emotional experiences.
The connectivity of “Internet of Things” will have a positive effect on consumers’ functional experiences.
3.2 Interactivity
IoT can link a typical daily activity to another situation or instruction; for example, removing a food from your refrigerator can create a reminder of an online purchase to replace it (Manches et al., 2015). Connecting objects through IoT raises several opportunities for the consumer, but also changes the way these objects interact with the environment (Christophe et al., 2011; Manches et al., 2015). Good interactivity between consumer and product can lead to satisfaction in consumer’s experience (Yaping et al., 2014). On the basis of this assertion, we formulated the following hypotheses:
The interactivity of “Internet of Things” will have a positive effect on consumers’ emotional experiences.
The interactivity of “Internet of Things” will have a positive effect on consumers’ functional experiences.
3.3 Sense of presence
Bian et al. (2016) found that connectable objects and electronic sensors can serve a number of public-related purposes, mainly prevention of diseases and management of public and environmental crisis. As internet and other relevant technologies continue to develop and mature, the number of solutions from market giants such as CISCO, IBM and others have made IoT a feasible option for large cities (Boulos and Al-Shorbaji, 2014; Barnor-Ahiaku, 2016). The sense of presence brought by the product stands out, resulting in a positive evaluation of the IoT device, thus offering a positive experience to consumers (Yaping et al., 2014). Hence, we formulated the following hypotheses:
“Internet of Things’” sense of presence will have a positive effect on consumers’ emotional experiences.
“Internet of Things’” sense of presence will have a positive effect on consumers’ functional experiences.
3.4 Intelligence
The fast development of connectable objects by IoT made it possible to link several smart objects through the internet, thus submitting more data for assessments. The forecast for the interconnection of these objects is automation in all fields of action, reducing the need for human interventions (Eisenberg and Fullerton, 2012; Hu et al., 2013; Bąk et al., 2015; Czarnecki and Deniziak, 2015; Kim, 2016). Intelligence is a feature that refers to the degree of automated functions, according to the operation of the IoT product. This product has sensors, memories, data processing and communication skills. If these attributes are complex to operate, they will reduce the efficiency of the IoT product, and consumers will consider it a low quality item (Yaping et al., 2014).
These authors, in their original article, state that the construct “intelligence” regarding the emotional experiences of consumers is the degree of automation during the product operation. Therefore, the greater the intelligence that IoT products provide to users, the less will be their concern about doing tasks/services/activities as they may carry out other activities. Thus, there is an exception with respect to H4a; based on the presented theory, it assumes a negative relationship between the constructs. Thereby:
IoT intelligence will have a negative effect on consumers’ emotional experiences.
IoT intelligence will have a positive effect on consumers’ functional experiences.
3.5 Convenience
Internet allows consumers to search and compare different products or services in distinct stores located anywhere in the world (Chang, 2013). The concept of online collective purchases is directly related to social networks, which is a structure formed by users and their interpersonal relationships. To improve the accuracy of location, IoT integrates several technologies such as internet, wireless, zigbee, Bluetooth, infrared, GRPS, 3G and 4G. A more efficient location can significantly affect convenience for activities inside or outside an environment (Chen et al., 2013). Therefore, convenience saves time and effort during purchase planning using the IoT product, and this product will receive a positive assessment from consumers (Yaping et al., 2014). On the basis of these assertions, we formulated the following hypotheses:
IoT convenience will have a positive effect on consumers’ emotional experiences.
IoT convenience will have a positive effect on consumers’ functional experiences.
3.6 Security
Engineers involved in the design of these connectable objects and sensors may not have the necessary qualification for data security problems (Peppet, 2014; Imgraben et al., 2014). Data security and privacy are concerns related to IoT as sensors can also harm the consumer’s well-being, if information and data are intercepted by a third party (Peppet, 2014).
The concept of trust is crucial, and affects several factors at the time of online transactions, including security and privacy issues. To reduce these barriers, online stores are building a circle of trust with their consumers, thus considering loyalty as crucial (Chang, 2013). The degree of security offered by the product that provides connectivity to IoT creates convenience for the consumer, and this is an important issue that affects the decision to buy the product (Yaping et al., 2014). Therefore, we defined the following hypotheses:
IoT security will have a positive effect on consumers’ emotional experiences.
IoT security will have a positive effect on consumers’ functional experiences.
3.7 Functional and emotional experiences and purchase intention
Classical theory assumes that consumers can appropriately assess the best path of their action, if they have as much information as possible about the product; however, they do not always check all possibilities for decision-making (Porto, 2010).
Consumers always establish relationships with a product that involve functional and emotional experiences. What can happen are different amounts of each. The functional experience of the product–customer relationship stems, to a large extent, from the satisfaction with the noticed usefulness of the IoT technology. In general, this satisfaction depends on the perceived quality, and is determined by the customer’s expectations regarding the product or service that will be offered to him, besides the perception of that same product or service after having consumed or used it.
However, there is a tendency for companies to strengthen the bonds of emotional experience. This means that a growing number of firms try to present their products with IoT technologies, to develop a relationship with the clients that goes beyond a simple transactional logic, by creating feelings of trust, esteem and closeness, reflecting cultural features such as customers’ values, which transcends the merely economic issue and functional satisfaction.
Hence, functional and emotional experiences, as well as purchase intention, are consumer’s expressions about the marketing, attributes and functionality of the IoT product (Yaping et al., 2014). Therefore, we suggest the following hypotheses:
Consumer’s functional experience will have a positive effect on purchase intention.
Consumer’s emotional experience will have a positive effect on purchase intention.
4. Method
This section presents the study design, comprising the research subject, its instruments, procedures and data treatment.
4.1 Participants, data collection, procedures, data treatment and validity
The population consisted of users of IoT products, to a greater or lesser degree. Data were collected by convenience, resulting in a non-probabilistic sampling technique, therefore limiting the generalization of results (Malhotra, 2014).
Hair et al. (2006) point out that the sample size for the pretest must comprise at least four and at most thirty individuals. On the other hand, Malhotra (2014) states that the pretest sample size should vary between 15 and 30 interviewees. To adapt the model to the Brazilian context, we used the process of reverse translation (checked by specialists in the field) and before applying the questionnaire, we submitted it to a pretest with 30 people, to check their understanding of the research instrument. Next step was to apply the survey to a bigger sample, and we considered 747 questionnaires in the final sample.
We collected data through a questionnaire applied in person, in different places such as colleges, streets, public parks, with an easy access to the target audience.
The questions were adapted from the model developed by Yaping et al. (2014), in which there are 18 different statements related to the influence of the attributes of IoT products, estimated through a Likert-type scale of five points, from 1 = totally disagree to 5 = totally agree; and on functional and emotional experiences and purchase intention, with 14 assertions measured by a seven-point Likert-type scale, with end points at 1 = totally disagree and 7 = totally agree.
According to Figure 1, we used the scale of influence of the attributes of IoT products of the original model (see Appendix). However, for the other constructs – “Functional Experience” (Behr and Becker, 2012; Ferraz et al., 2014), “Emotional Experience” (Venkatesh and Bala, 2008; Wu, 2011) and “Purchase Intention” (Chandran and Morwitz, 2005) – we adapted other scales related to their application, with due care to keep the orientation proposed by the authors of the original paper. There are also demographic issues and others regarding the use of IoT products. Questionnaires were completed by the respondents themselves, in the researchers’ presence, after a brief presentation of the study.
We chose the model based on partial least squares, or PLS. After finding a non-normal distribution in the data sets of several sample variables (Ringle et al., 2014), we decided to use the PLS Structural Equation Modeling (PLS–SEM) approach, using the Smart-PLS software.
Figure 1 shows the proposed model with the additional constructs that were included for the identification of functional and emotional experiences, as well as purchase intention. The next section presents the result analysis.
5. Result analysis
Here, we present the analyses regarding the application of the method previously described on the latent variables, and the implications of the results on the formulated hypotheses.
5.1 Respondents’ attributes
The descriptive analysis made possible the contextualization of the socioeconomic reality of participants. Table II shows details of gender, age group, family income and educational level.
According to the demographic analysis of survey respondents, more than half (n = 378; 50.60 per cent) belong to the female gender and even being a non-probabilistic sample, its features are consistent with the target audience that uses IoT products (smartphones, tablets and smartwatches): 71.35 per cent (n = 533) of the respondents are under 25 years old, 71.08 per cent (n = 531) are undergraduate, and 31.59 per cent (n = 235) have a family income between R$4,427.36 and R$8,695.88. That is, according to Brazilian Association of Research Companies (ABEP), most respondents belong to the socioeconomic stratum B2. Genders had similar frequencies in all socioeconomic strata, and the female audience stands out in most strata.
The research showed that 96.21 per cent (n = 719) of the sample use smartphones more frequently, followed by notebooks (n = 493; 65.99 per cent), PCs (n = 378; 50.60 per cent), SmartTVs (n = 277; 37.08 per cent), tablets (n = 208; 27.84 per cent) and smartwatches (n = 61; 8.16 per cent).
The average amount spent on IoT products was R$1,986.95, and they bought, on average, 1.67 products in the previous year, with the average value and number of products being higher for the female gender (R$2,103.99 and 1.74 products); that is, considering our sample, the female gender bought more IoT products than the male.
The average frequency of IoT products used in the same day is 2.43, and most people use them at home (n = 702; 93.97 per cent), followed by workplace (n = 362; 48.46 per cent), public places (n = 345, 46.18 per cent), leisure places (n = 264; 35.34 per cent), cars (n = 117; 15.6 per cent) and others (n = 46; 6.15 per cent).
Regarding geofencing, 60.24 per cent (n = 450) of the respondents consider it the most important technology. Beacons are considered by 24.63 per cent (n = 184), and these are the least important among the presented technologies; instead, when considering income, beacons overcome wearable technology in socioeconomic stratum C1.
5.2 Assumptions for the use of structural equation modeling and analysis of the structural model
Regarding the model’s predictor variables, we observed the lack of multicollinearity in data distribution as all variance inflation factors (VIF) values were below 5 (lowest = 1.046 and highest = 1.503). All indicators test p-values were significant (p < 0.01). Likewise, there was non-normality in the distribution of the dependent variable data, as proved by observing the significant Kolmogorov–Smirnov (KS) Z statistics (p < 0.05), presented in Appendix. This result supported the decision to use the correlation matrix, which is the estimator of PLS (Ringle et al., 2014). The following step was to examine the convergent and discriminant validities, as shown in Tables III and IV.
For this model, AVEs varied between 0.500 and 0.721, meeting the criteria for the existence of convergent validity. Composite reliabilities varied between 0.759 and 0.912, which is considered “very good” (Ringle et al., 2014).
Values above 0.60 are considered appropriate. This median result indicates that the internal consistency of the used scales needs improvement (Ringle et al., 2014). However, the result does not invalidate our study as the coefficient is very sensitive to the sample size (Peterson, 1994; Helms, 1999). On the other hand, the model’s determination coefficients (R2 value) show that it has a higher accuracy and predictive relevance for the emotional and functional experiences, and lower for the purchase intention (Malhotra, 2014).
AVEs were higher or equal to the squared correlation between the factors, as presented in Table IV, through the definitive Fornell and Larcker (1981) criterion. All factorial loads for each indicator had values above 0.5, after the exclusion of variables inter01, int03 e funcexp04, for the model’s adjustment.
After the first iteration in Smart–PLS software, we present the results of the factorial loads of each latent variable. All had values above 0.5 (Figure 2), after removing three indicators to adjust the model. The measuring scale in structural equation modeling used in this study is reflexive. Hence, the direction of causality starts from the latent variable to the indicators (Hair et al., 2014).
Building the measurement model also involved constructs’ validation, based on the check of reliability and significance between the variables, through confirmatory factorial analysis (Figure 2), according to the final model achieved in this study. The proposed model was estimated by using the bootstrapping technique, comparing the original sample with the samples created by this technique. Other 1.000 samples were generated, and we performed Student’s t test, as shown in Table V.
The results from the analysis of path significance indicated that most had no difference between the original sample and the subsamples created by the statistical technique with the critical limits for Student’s t test, which allows the analysis of correlation/regression coefficients to be equal to zero (Hair et al., 2006). We considered Student’s t-values significant for p < 0.05 given that the critical limits for this test were p < 0.05 = 1.96 and p < 0.01 = 2.57 (bilateral test = level of significance = P – Type I Error). Therefore, we present the analyses of each of the paths of the corresponding research hypotheses.
The causal relationship of the path “Connectivity” and “Emotional experience”, with original load β = −0.038 and t test = 1.148, was not significant owing to the p value < 5 per cent, thus rejecting H1a (+). In general, respondents believe that IoT products enable extensive connections, which indicates that the attribute “Connectivity”, which measures the degree of proximity of interconnection of products with IoT, does not affect their emotional experience.
Next, the path from “Connectivity” to “Functional experience”, with original load β = 0.196 and t test = 6.060, was significant, showing that connectivity has an influencing factor on the functional experiences of individuals. Hence, those that live in an environment of abundant information, with the increase in sensors that monitor their daily activities, learned to measure, track and control from a distance the connectable objects (intelligent objects) that allowed extensive connections, thus confirming H1b (+).
The path tested by H2a (+), between “Interactivity” and “Emotional experience”, presented load β = 0.063 and t test = 1.695, indicating a significant relationship at 5 per cent level. The IoT products are changing their use interfaces, substituting the mouse and keyboard with more objective forms of interactivity, such as touchscreens and gesture recognition (Manches et al., 2015). In addition, more than half of the users considered geofencing as the most important as it requires greater interactivity to facilitate and assist in their locomotion.
The path from “Interactivity” to “Functional experience”, with original load β = 0.068 and t test = 1.973, presented a significant relationship, with p < 5 per cent and confirming H2b (+). This shows that respondents consider interactivity as an attribute that affects their functional experience by transmitting information between convenient products. IoT products brought a remote communication, which has a direct response. Most respondents have smartphones and consider geofencing as a major technology; thus, product interactivity becomes essential for satisfying their functional experience (Porto, 2010).
The relationship between “Sense of presence” and “Emotional experience” was significant, as H3a (+) had predicted (β = 0.478, t = 15.002; p < 0.01). For Yaping et al. (2014), it is a positive feature in the emotional experience of satisfaction with the IoT product, thus becoming an affective component (Porto, 2010). Most respondents use connectable products at home and at work, which indicates that the sense of presence is a relevant feature.
The path from “Sense of presence” to “Functional experience” was not proven (β = 0.025, t = 0.802, p > 0.10), thus rejecting H3b (+). Although the sense of presence, whose examples are connectable objects and electronic sensors that serve several purposes related to the audience (Boulos and Al-Shorbaji, 2014), has a significant relationship with emotional experience, it does not affect the functional experience as it is not a feature that individuals look for when they search a product and buy it.
In addition, the causal relationship of the path from “Intelligence” to “Emotional experience” was significant, with high load β = −0.081 and t test = 2.624, confirming H4a (−). This means that in respondents’ evaluation, the IoT product is easy to operate and its degree of automation negatively affects their emotional experience (Yaping et al., 2014). The world is a high intelligence environment in parallel with a universe of monitors and sensors that interact and provide feedback to users. To a large extent, people are getting used to wearable technologies (Eisenberg and Fullerton, 2012).
The path from “Intelligence” to “Functional experience” for H4b (+) got the highest value for t test = 16.112 and a high original load β = 0.471. Hu et al. (2013) observe that sensors, devices and people connected to internet create countless amounts of data that are digitally available, and the forecast for interconnection of these connectable objects will be automation in all fields, reducing the need for human interventions (Kim, 2016).
In the case of the path from “Convenience” to “Emotional experience”, with original load β = 0.200 and t test = 6.307, there is a positive effect between these constructs, as predicted by H5a (+). According to Chang (2013), IoT products allow consumers to search and compare different products or services in distinct stores, located anywhere. This increases time saving and planning efforts for their purchases, and most respondents said that they use these products, having spent an average of R$2,000.00 in the previous year.
The path from “Convenience” to “Functional experience” had original load β = 0.169 (t = 5.052, p < 0.01), indicating a high relationship between the latent variables of H5b (+). This shows that respondents value the degree of effort and time saving of the functional experience of their IoT product (Yaping et al., 2014). Thus, they recognize that the IoT integrates different technologies (Chen et al., 2013). More than half of them consider geofencing as a major technology that facilitates their lives, meaning that a more efficient location can significantly affect the convenience for activities inside or outside an environment.
“Security” and “Emotional experience” paths, with original load β = 0.211 and t test = 6.332, showed a significant relationship, with p value> 1 per cent. Sensors may harm consumers’ well-being (Peppet, 2014) as it is probable that the increasing popularity of connectable objects will lead criminals to focus on these devices. Besides validating H6a (+), this confirms that information such as passwords and private data stored in these devices may be targets of malware (Imgraben et al., 2014).
The relationship between “Security” and “Functional experience” was not significant (β = −0.027; t = 0.955, p > 0.10), so that H6b (+) was rejected. Therefore, individuals do not feel the effects of product safety during their use, which is a necessary feature for functional experience. This lack of significance would be a concern regarding the security and privacy of data collected from various connectable devices, often without the consumer’s consent (Imgraben et al., 2014; Peppet, 2014).
The relationship between “Functional experience” and “Purchase intention” got load β = 0.095 (t = 2.346; p < 5 per cent), indicating that this path is significant, as predicted in H7 (+). Thus, when individuals have the intention to buy, they evaluate the functionality of the IoT product. Cognition in decision-making is idealized through direct observation, experience with products, information and inference processes; in short, if a product of a certain category is similar to another of the same category (Roman et al., 2015).
Finally, H8 (+) indicates that the relationship between “Emotional experience” and “Purchase intention” was significant, with load β = 0.326 (t = 9.786; p < 1 per cent). Therefore, individuals who consider that the product does not require much effort to be used, feel that their subjective experiences, related to an object, person or situation, are significant for the intention to buy. In addition, consumers learn to purchase products whose cognitive adjustments with environmental and social factors relate to their past experiences (Roman et al., 2015).
6. Conclusions
This study was an attempt to deepen the research on the influence of the attributes of the IoT products on the functional and emotional experiences and consumers’ purchase intention. By adapting the model created by Yaping et al. (2014), we showed that most of the constructs are applicable. This result shows the importance of the attributes of IoT products at the time of purchase.
The research allowed the confirmation of 11 of the 14 hypotheses presented. We can say that the constructs interactivity, sense of presence, intelligence, convenience and security have a significant effect on the emotional experience, and that connectivity, interactivity, intelligence and convenience have a positive effect on the functional experience.
By analyzing the effects on the intention to buy, we showed that both are significant, but the emotional experience has a greater influence on purchase intention. From the paths proposed in this model, for the selected sample, emotional experience is the one with greatest influence on purchase intention; hence, by all different levels of significance, the emotional experience is more intense and noticeable than the functional experience.
The results achieved in our research were distinct from those of the original article by Yaping et al. (2014), who used a sample of Chinese individuals, and whose result presented the functional experience as the one that most affects purchase intention. A possible explanation is the different cultural features of the two countries. For the Chinese audience, the functional value relates to the fact that consumers are more aware of their expenses and investments, which are led by a “utilitarian” attitude toward IoT resources, while Brazilians are driven by “emotions”.
We can say that emotional experience was the closest construct to respondents’ conception about judgments related to previous experiences because it is independent and precedes perceptions and cognitive actions adopted in purchase intention. Thus, respondents in our research tend to make decisions, at the time of purchase, based on past experiences. Functional experience is also important for the intention to buy an IoT product as its performance, usability and the whole set of attributes are essential for purchase intention.
As managerial recommendations, we suggest that companies that develop IoT products should consider the attributes with the lowest significance in our study, and check consumers’ needs to know what they think about these attributes.
In general, we observe a greater aggressiveness of these companies in creating new products for a specific market, which experiences rapid changes and is very popular among consumers. These fast changes are increasingly coming from highly informed, connected consumers, with new needs. Our sample consisted mainly of young college students prone to use technological products adherent to IoT.
Figures
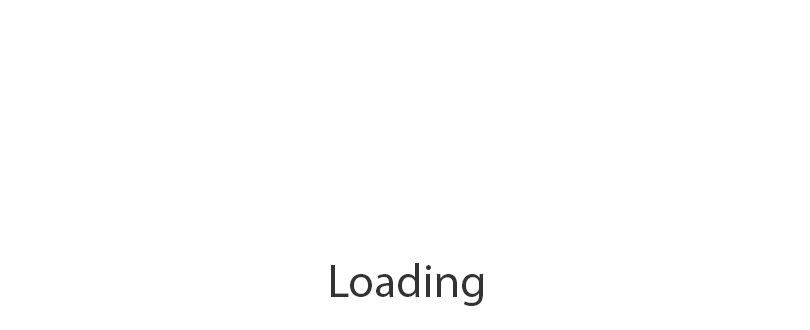
Figure 2.
Convergent validity model adapted from Yaping et al. (2014)
Internet of things’ applications
Applications | Description | References |
---|---|---|
Beacons | Precise location of objects in closed environments. Example: when a consumer enters a store, he is informed about special offers | Mautz (2009) Jae-Yoon et al. (2016) |
Geofencing | Practice of using GPS to define a geographic border (liberation or barrier defined by the manager). Ex: an “internet of things” product recognizes when user leaves home, location of his car, etc. | Fisher and Raquet (2011) |
Wearable technology | Wearable computing or wearable technology, allows the measure of pulse and body pressure, alerts and notifications from connectable objects. Ex: smartwatches, smartclothes, etc. | Chalfen (2014) Kaewkannate and Kim (2016) |
Machine learning | Computers’ ability to learn without being explicitly programmed. Ex: spellchecker, suggestion of friends (social networks), etc. | Qiu et al. (2016) Fernández-Manzano et al. (2016) |
Respondents’ demographic attributes
Attributes | Type | N | Frequency (%) |
---|---|---|---|
Gender | Male | 378 | 50.60 |
Female | 369 | 49.40 | |
Total | 747 | 100 | |
Age group | <20 years | 288 | 38.55 |
From 21 to 25 years | 245 | 32.80 | |
From 26 to30 years | 102 | 13.65 | |
From 31 to 35 years | 40 | 5.35 | |
From 36 to 40 years | 27 | 3.61 | |
From 41 to 45 years | 17 | 2.28 | |
From 46 to 50 years | 10 | 1.34 | |
>51 years | 18 | 2.41 | |
Total | 747 | 100 | |
Family income | Less than R$ 63,978 | 6 | 0.80 |
From R$63,978 to R$144,6 24 | 46 | 6.16 | |
From R$144,624 to R$240,901 | 84 | 11.24 | |
From R$240,901 to R$442,736 | 212 | 28.38 | |
From R$442,736 to R$869,588 | 236 | 31.59 | |
From R$869,588 to R$2,027,256 | 140 | 18.74 | |
More than R$2,027,256 | 23 | 3.08 | |
Total | 747 | 100 | |
Education | High school incomplete | 10 | 1.34 |
Completed high school | 42 | 5.62 | |
Attending undergraduate course | 531 | 71.08 | |
Completed undergraduate course | 118 | 15.80 | |
Attending postgraduate course | 22 | 2.95 | |
Completed postgraduate course | 24 | 3.21 | |
Total | 747 | 100 |
Convergent validity
Indicators | Average variance extracted (AVE) > 0.50 | Composite reliability (CR) > 0.70 | Cronbach’s alpha > 0.60 | R2 | No. of items |
---|---|---|---|---|---|
Connectivity | 0.518 | 0.759 | 0.540 | – | 3 |
Interactivity | 0.631 | 0.772 | 0.520 | – | 2 |
Sense of presence | 0.525 | 0.776 | 0.556 | – | 3 |
Intelligence | 0.621 | 0.759 | 0.510 | – | 2 |
Convenience | 0.548 | 0.783 | 0.584 | – | 3 |
Security | 0.538 | 0.768 | 0.564 | – | 3 |
Emotional experience | 0.517 | 0.841 | 0.761 | 0.435 | 5 |
Functional experience | 0.500 | 0.790 | 0.649 | 0.468 | 4 |
Purchase intention | 0.721 | 0.912 | 0.871 | 0.134 | 4 |
Model adjusted to the number of indicators that were part of the study
Discriminant validity: Fornell and Larcker’s criterion
Latent variables | (1) | (2) | (3) | (4) | (5) | (6) | (7) | (8) | (9) |
---|---|---|---|---|---|---|---|---|---|
(1) Connectivity | 0.720 | ||||||||
(2) Convenience | 0.354 | 0.740 | |||||||
(3) Emotional experience | 0.202 | 0.342 | 0.719 | ||||||
(4) Functional experience | 0.462 | 0.390 | 0.173 | 0.697 | |||||
(5) Intelligence | 0.374 | 0.266 | 0.136 | 0.607 | 0.788 | ||||
(6) Purchase intention | 0.161 | 0.118 | 0.333 | 0.160 | 0.072 | 0.849 | |||
(7) Interactivity | 0.426 | 0.353 | 0.349 | 0.353 | 0.288 | 0.214 | 0.795 | ||
(8) Security | 0.206 | 0.138 | 0.369 | 0.183 | 0.269 | 0.141 | 0.180 | 0.733 | |
(9) Sense of presence | 0.269 | 0.262 | 0.597 | 0.246 | 0.215 | 0.230 | 0.453 | 0.309 | 0.725 |
The diagonal in italic numbers shows AVE square roots
Analysis of path significance
Hypothesis | Path | (β) | Bootstrapping 1.000 | Standardized error | t-test | p values | Sig. |
---|---|---|---|---|---|---|---|
Direct effects | |||||||
H1a (+) | Connectivity > Emotional experience | −0.038 | −0.037 | 0.033 | 1.150 | 0.251 | N.S. |
H1b (+) | Connectivity > Functional experience | 0.196 | 0.198 | 0.032 | 6.06 | 0.000 | * |
H2a (+) | Interactivity > Emotional experience | 0.063 | 0.061 | 0.037 | 1.695 | 0.090 | ** |
H2b (+) | Interactivity > Functional experience | 0.068 | 0.069 | 0.035 | 1.973 | 0.049 | ** |
H3a (+) | Sense of presence > Emotional experience | 0.478 | 0.479 | 0.032 | 15.002 | 0.000 | * |
H3b (+) | Sense of presence > Functional experience | 0.025 | 0.026 | 0.032 | 0.802 | 0.423 | N.S. |
H4a (-) | Intelligence > Emotional experience | −0.081 | −0.082 | 0.031 | 2.624 | 0.009 | * |
H4b (+) | Intelligence > Functional experience | 0.471 | 0.473 | 0.029 | 16.112 | 0.000 | * |
H5a (+) | Convenience > Emotional experience | 0.200 | 0.201 | 0.032 | 6.307 | 0.000 | * |
H5b (+) | Convenience > Functional experience | 0.169 | 0.166 | 0.033 | 5.052 | 0.000 | * |
H6a (+) | Security > Emotional experience | 0.211 | 0.212 | 0.033 | 6.332 | 0.000 | * |
H6b (+) | Security > Functional experience | −0.027 | −0.026 | 0.028 | 0.955 | 0.34 | N.S. |
H7(+) | Emotional experience > Purchase intention | 0.326 | 0.326 | 0.033 | 9.786 | 0.000 | * |
H8(+) | Functional experience > Purchase intention | 0.095 | 0.096 | 0.040 | 2.346 | 0.019 | ** |
Significance:
p < 0.01;
p < 0.05; N.S. = Not significant
Identification of latent variables, indicators, assertions description, normality and multicollinearity test
Latent variable | Indicators | Assertions | K–S test | p-value | VIF |
---|---|---|---|---|---|
Connectivity Yaping et al. (2014) |
con01 | IoT products are those that connect with each other | 5.147 | 0.000 | 1.259 |
con02 | IoT products communicate satisfactorily with each other | 5.378 | 0.000 | 1.490 | |
con03 | IoT products allow extensive connections | 5.571 | 0.000 | 1.539 | |
Interactivity Yaping et al. (2014) |
inter01 | With IoT products, I can easily transfer relevant information to other products | 5.848 | 0.000 | 1.299 |
inter02 | IoT products can send me timely feedback with relevant information | 5.343 | 0.000 | 1.498 | |
inter03 | Information communication between me and the IoT product is very convenient | 5.342 | 0.000 | 1.568 | |
Sense of presence Yaping et al. (2014) |
sens01 | When using an IoT product, I have the impression that I am personally communicating with it | 4.767 | 0.000 | 1.545 |
sens02 | The sensation of communication between me and the IoT product is quite real | 5.001 | 0.000 | 1.435 | |
sens03 | Using an IoT product makes me feel very enthusiastic | 5.083 | 0.000 | 1.546 | |
Intelligence Yaping et al. (2014) |
int01 | The IoT product does not require me to perform complex configurations to complete its tasks | 5.551 | 0.000 | 1.288 |
int02 | The IoT product is simple to operate | 6.084 | 0.000 | 1.997 | |
int03 | The IoT product does not require a complex reasoning process | 5.221 | 0.000 | 1.213 | |
Convenience Yaping et al. (2014) |
conv01 | The IoT product makes my life easy | 7.702 | 0.000 | 1.571 |
conv02 | The IoT product gives me comfort | 7.259 | 0.000 | 1.497 | |
conv03 | The IoT product increases my efficiency | 6.100 | 0.000 | 1.414 | |
Security Yaping et al. (2014) |
sec01 | The use of the IoT product is very safe | 5.054 | 0.000 | 1.526 |
sec02 | The use of the IoT product is very stable | 5.696 | 0.000 | 1.542 | |
sec03 | I am not worried about the security issues related to the IoT product | 5.346 | 0.000 | 1.306 | |
Emotional experience Ferraz et al. (2014); Behr and Becker (2012) |
emoexp01 | When I use an IoT product I feel happy | 5.157 | 0.000 | 1.889 |
emoexp02 | When I use an IoT product I feel powerful | 5.164 | 0.000 | 1.612 | |
emoexp03 | Using an IoT product increases my self-esteem | 5.133 | 0.000 | 1.586 | |
emoexp04 | When I use an IoT product I feel emotionally satisfied | 5.987 | 0.000 | 1.446 | |
emoexp05 | When I use an IoT product I experiment strong emotions | 5.566 | 0.000 | 2.149 | |
Functional experience Wu (2011); Venkatesh and Bala (2008) |
funcexp01 | The IoT product interface is friendly | 7.057 | 0.000 | 1.610 |
funcexp02 | The procedure for using the IoT product is understandable | 7.06 | 0.000 | 1.624 | |
funcexp03 | It’s easy to learn how to use the IoT product | 6.593 | 0.000 | 1.638 | |
funcexp04 | It’s easy to use the IoT product | 5.633 | 0.000 | 1.352 | |
funcexp05 | The IoT product does not require much effort to be used | 5.659 | 0.000 | 1.602 | |
Purchase intention Chandran and Morwitz (2005) | PI01 | How much are you prone to buying an IoT product, if it’s on sale? | 4.155 | 0.000 | 2.914 |
PI02 | If the IoT product is on sale, how likely is it that you will buy it? | 4.403 | 0.000 | 3.021 | |
PI03 | How sure are you of buying an IoT product? | 3.673 | 0.000 | 2.779 | |
PI04 | What is the chance of buying an IoT product? | 4.113 | 0.000 | 2.584 |
Appendix
References
Ashton, K. (2009), “That internet of things thing”, RFID Journal, Vol. 22 No. 7, pp. 97-114.
Atzori, L., Iera, A. and Morabito, G. (2010), “The internet of things: a survey”, Computer Networks, Vol. 54 No. 15, pp. 2787-2805.
Bąk, S., Czarnecki, R. and Deniziak, S. (2015), “Synthesis of real-time cloud applications for internet of things”, Turkish Journal of Electrical Engineering & Computer Sciences, Vol. 23 No. 3, pp. 913-929, doi: 10.3906/elk-1302-178.
Barnor-Ahiaku, E. (2016), “Exploring the use of smartphones and tablets by medical house officers in Korle-Bu Teaching hospital”, Ghana Medical Journal, Vol. 50 No. 1, pp. 50-56, doi: 10.4314/gmj.v50i1.8.
Behr, M. and Becker, M. (2012), “Scales for experiencing emotions: awareness, appraisal and regulation of one’s own emotions”, Hellenic Journal of Psychology, Vol. 9 No. 3, pp. 278-303.
Bian, J., Yoshigoe, K., Hicks, A., Yuan, J., He, Z., Xie, M. and Modave, F. (2016), “Mining twitter to assess the public perception of the internet of things”, Plos One, Vol. 11 No. 7, pp. 1-14, doi: 10.1371/journal.pone.0158450.
Boulos, M.K. and Al-Shorbaji, N.M. (2014), “On the internet of things, smart cities and the WHO healthy cities”, International Journal of Health Geographics, Vol. 13 No. 1, pp. 10-15, doi: 10.1186/1476-072X-13-10.
Chalfen, R. (2014), “Your panopticon or mine?’ Incorporating wearable technology’s glass and GoPro into visual social science”, Visual Studies, Vol. 29 No. 3, pp. 299-310, doi: 10.1080/1472586X.2014.941547.
Chandran, S. and Morwitz, V.G. (2005), “Effects of participative pricing on consumers’ cognitions and actions: a goal theoretic perspective”, Journal of Consumer Research, Vol. 32 No. 2, pp. 249-259.
Chang, C. (2013), “What Otaku consumers care about: the factors influential to online purchase intention”, AIP Conference Proceedings, American Institute of Physics, Melville, NY, Vol. 1558 No. 1, pp. 450-454, doi: 10.1063/1.4825523.
Chen, Z., Xia, F., Huang, T., Bu, F. and Wang, H. (2013), “A localization method for the internet of things”, The Journal of Supercomputing, Vol. 63 No. 3, pp. 657-674, doi: 10.1007/s11227-011-0693-2.
Christophe, B., Boussard, M., Lu, M., Pastor, A. and Toubiana, V. (2011), “The web of things vision: things as a service and interaction patterns”, Bell Labs Technical Journal, Vol. 16 No. 1, pp. 55-61, doi: 10.1002/bltj.20485.
Czarnecki, R. and Deniziak, S. (2015), “Embedded real-time HTTP server”, International Journal of Computer Network and Information Security, Vol. 7 No. 5, pp. 1-8, doi: 10.5815/ijcnis.2015.05.01.
Eisenberg, M.B. and Fullerton, S.P. (2012), “ED and INFO 2052: oh, the places you’ll go!”, Information Services & Use, Vol. 32 Nos 3/4, pp. 103-115.
Fernández-Manzano, E., Neira, E. and Clares-Gavilán, J. (2016), “Data management in audiovisual business: Netflix as a case study”, El Profesional De La Información, Vol. 25 No. 4, pp. 568-576, doi: 10.3145/epi.2016.jul.06.
Ferraz, I.R., Barreto, I.F. and Menezes, V.C. (2014), “Motivações do jovem consumidor de produtos de luxo no Brasil”, Anais Do 17° SemeAd – Seminários Em Administração, FEA/USP. São Paulo, SP.
Fisher, K.A. and Raquet, J.F. (2011), “Precision position, navigation, and timing without the global positioning system”, Air & Space Power Journal, Vol. 25 No. 2, pp. 24-33.
Fornell, C. and Larcker, D.F. (1981), “Evaluating structural equation models with unobservable variables and measurement error”, Journal of Marketing Research, Vol. 18 No. 1, pp. 39-50.
Galegale, G.P., Siqueira, E., Silva, C.B.H. and Souza, C.A. (2016), “Internet das coisas aplicada a negócios – um estudo bibliométrico”, Journal of Information Systems and Technology Management, Vol. 13 No. 3, pp. 423-438, doi: 10.4301/S1807-17752016000300004.
Hair, J.R., Black, W.C., Babin, B.J., Anderson, R.E. and Tatham, R.L. (2006), Multivariate Data Analysis, 6a. ed., Pearson Prentice Hall, Upper Saddle River, NJ.
Hair, J.F., Hult, G.T.M., Ringle, C. and Sarstedt, M. (2014), A Primer on Partial Least Squares Structural Equation Modeling (PLS-SEM), Sage Publications, Thousand Oaks, CA, doi: 10.1080/1743727X.2015.1005806.
Hancock, B. and Hancock, L.N. (2016), “Somebody’s watching: the ever-growing internet of things”, Phi Kappa Phi Journal (National Forum), Vol. 96 No. 3, pp. 12-15.
Helms, J.E. (1999), “Another Meta-analysis of the white racial identity scale’s cronbach alphas: Implications for validity”, Measurement and Evaluation in Counseling and Development, Vol. 32 No. 3, pp. 122-137.
Hu, J., van der Vlist, B., Niezen, G., Willemsen, W., Willems, D. and Feijs, L. (2013), “Designing the internet of things for learning environmentally responsible behaviour”, Interactive Learning Environments, Vol. 21 No. 2, pp. 211-226, doi: 10.1080/10494820.2012.705858.
Imgraben, J., Engelbrecht, A. and Choo, K.R. (2014), “Always connected, but are smart mobile users getting more security savvy? A survey of smart mobile device users”, Behaviour & Information Technology, Vol. 33 No. 12, pp. 1347-1360, doi: 10.1080/0144929X.2014.934286.
Jae-Yoon, K., Se-Hoon, Y., Yong-Hwan, S. and Sang-Kook, H. (2016), “High-resolution indoor positioning using light emitting diode visible light and camera image sensor”, IET Optoelectronics, Vol. 10 No. 5, pp. 184-192, doi: 10.1049/iet-opt.2015.0073.
Kaewkannate, K. and Kim, S. (2016), “A comparison of wearable fitness devices”, BMC Public Health, Vol. 16 No. 1, pp. 1-16, doi: 10.1186/s12889-016-3059-0.
Kim, S. (2016), “Behavioral learning game for socio-physical IoT connections”, EURASIP Journal on Wireless Communications and Networking, Vol. 2016 No. 1, pp. 1-11, doi: 10.1186/s13638-016-0521-8.
Malhotra, N.K. (2014), Essentials of Marketing Research: A Hands-on Orientation, Pearson Prentice Hall, Upper Saddle River, NJ.
Manches, A., Duncan, P., Plowman, L. and Sabeti, S. (2015), “Three questions about the internet of things and children”, TechTrends, Vol. 59 No. 1, pp. 76-83, doi: 10.1007/s11528-014-0824-8.
Mautz, R. (2009), “Overview of current indoor positioning systems”, Geodezija Ir Kartografija, Vol. 35 No. 1, pp. 18-22.
Peppet, S.R. (2014), “Regulating the internet of things: first steps toward managing discrimination, privacy, security, and consent”, Texas Law Review, Vol. 93 No. 1, pp. 85-178.
Peterson, R.A. (1994), “A Meta-analysis of cronbach’s coefficient alpha”, Journal of Consumer Research, Vol. 21 No. 2, pp. 381-391.
Porter, M.E. and Heppelmann, J.E. (2014), “How smart, connected products are transforming competition”, Harvard Business Review, Vol. 92 No. 11, pp. 64-88.
Porto, R.B. (2010), “Atitude do consumidor: Estrutura dos modelos teóricos”, Revista Brasileira De Marketing, Vol. 9 No. 2, pp. 41-58, doi: 10.5585/remark.v9i2.2145.
Qiu, J., Wu, Q., Ding, G., Xu, Y. and Feng, S. (2016), “A survey of machine learning for big data processing”, EURASIP Journal on Advances in Signal Processing, Vol. 2016 No. 1, pp. 1-16, doi: 10.1186/s13634-016-0355-x.
Ringle, C.M., Silva, D. and Bido, D.D.S. (2014), “Modelagem de equações estruturais com utilização do SmartPLS”, REMark – Revista Brasileira De Marketing, Vol. 13 No. 2, pp. 56-73.
Rivera, J. and Meulen, R.V. (2014). “Gartner says 4.9 billion connected things will be in use in 2015”, 11 November, available at: www.gartner.com/newsroom/id/2905717
Roman, T., Vasilache, S., Maxim, E. and Manolica, A. (2015), “Factors that influence the behaviour of consumers of smartphone services in multinational companies”, Current Science, Vol. 109 No. 6, pp. 1038-1046, doi: 10.18520/v109/i6/1038-1046.
Said, O. and Masud, M. (2013), “Towards internet of things: Survey and future vision”, International Journal of Computer Networks, Vol. 5 No. 1, pp. 1-17.
Sánchez-Alcón, J., López-Santidrián, L. and Martínez, J. (2015), “Solución Para garantizar la privacidad en internet de las cosas”, El Profesional De La Información, Vol. 24 No. 1, pp. 62-70, doi: 10.3145/epi.2015.ene.08.
Sánchez-Alcón, J., López, L., Martínez, J.F. and Rubio-Cifuentes, G. (2015), “Trust and privacy solutions based on holistic service requirements”, Sensors, Vol. 16 No. 1, pp. 1-38, doi: 10.3390/s16010016.
Venkatesh, V. and Bala, H. (2008), “Technology acceptance model 3 and a research agenda on interventions”, Decision Sciences, Vol. 39 No. 2, doi: 10.1111/j.1540-5915.2008.00192.x.
Wortmann, F. and Flüchter, K. (2015), “Internet of things”, Business & Information Systems Engineering, Vol. 57 No. 3, pp. 221-224.
Wu, W. (2011), “Developing an explorative model for SaaS adoption”, Expert Systems with Applications, Vol. 38 No. 12, pp. 15057-15064.
Yaping, C., Xuebing, D. and Wei, S. (2014), “Influence of characteristics of the internet of things on consumer purchase intention”, Social Behavior and Personality: An International Journal, Vol. 42 No. 2, pp. 321-330, doi: 10.2224/sbp.2014.42.2.321.
Younes, F., Halawi, G., Jabbour, H., El Osta, N., Karam, L., Hajj, A. and Rabbaa Khabbaz, L. (2016), “Internet addiction and relationships with insomnia, anxiety, depression, stress and self-esteem in university students: a cross-sectional designed study”, Plos One, Vol. 11 No. 9, pp. 1-13, doi: 10.1371/journal.pone.0161126.